Intelligent decision system with predictive model in smart environments
Ευφυές σύστημα απόφασης με μοντέλο πρόβλεψης σε έξυπνα περιβάλλοντα
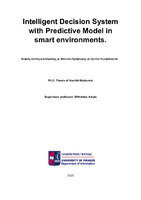
Doctoral Thesis
Συγγραφέας
Matzavela, Vasiliki
Ματζαβέλα, Βασιλική
Ημερομηνία
2023Επιβλέπων
Alepis, EfthimiosΑλέπης, Ευθύμιος
Προβολή/ Άνοιγμα
Λέξεις κλειδιά
Artificial intelligence ; Machine learning ; Predictive algorithms ; Intelligent decision tree learning ; Data mining ; Binary tree ; Mathematic assessment ; Τεχνητή νοημοσύνη ; Μηχανική μάθηση ; Προβλεπτικοί αλγόριθμοι ; Ευφυής μάθηση δέντρων απόφασης ; Εξόρυξη δεδομένων ; Δυαδικό δέντρο ; Μαθηματική αξιολόγησηΠερίληψη
In this Ph.D. thesis a novel approach of digital education and hybrid education that
presents effective individualized learning, adapting all knowledge domains to the
learner’s needs and path through a predictive model supported by a decision binary
tree, is presented. The thesis is based on papers of other researchers that were
published in international journals, as well as on the author's papers, that were
published in valid journals with a plethora of citations.
The presented approach models the learning path in m-learning environments or how
the student’s knowledge can be increased. In particular, it performs user modeling
by dynamically identifying and updating the student’s knowledge level for all the
concepts of the domain knowledge. Its operation is based on AI and a predictive
model of learners' assessment(Fig. 1).
The presented novel approach was fully implemented and evaluated. Notably, an original system for digitalized m-learning was developed, based on the
programming language JAVA, and the resulting app is called D-Quest. The specific
knowledge domain that was chosen, was Mathematics since it has a connection with
computer science. Supplementally, informatics and Mathematics have the best knowledge
background and embedded implementation combination. Therefore, it is suitable for the
implementation and evaluation of the thesis issue. D-Quest incorporates the
presented student assessment modeling approach. Thereby, the predictive model of the
decision tree visualizes dynamically the path for a new domain concept that is
completely unknown to the learner. Furthermore, it recognizes when a previously
known domain concept has been completely or partly forgotten by the learner.
The application of this approach is not limited to m-learning environments, but it
can also be used in other systems with changeable user states, such as
e-shops and smart cities, where consumers’ preferences change over time and affect
one another. Consequently, the approach constitutes a novel predictive model for the
evaluation of big data sets, which offers a dynamic adaptation to users’ needs and
preferences for intelligent systems.