Μηχανική μάθηση στην πρόβλεψη της τιμής ενοικίασης Airbnb στο Άμστερνταμ
Machine learning prediction of Amsterdam Airbnb prices
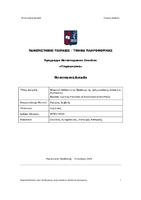
Προβολή/ Άνοιγμα
Λέξεις κλειδιά
Μηχανική μάθηση ; ΠρόβλεψηΠερίληψη
Σε αυτή τη διατριβή, διερευνάται η χρήση και σύγκριση μοντέλων μηχανικής μάθησης για την πρόβλεψη της τιμής μιας καταχώρισης Airbnb. Πιο συγκεκριμένα, συλλέχτηκε ένα σύνολο δεδομένων από καταχωρίσεις της Airbnb στο Amsterdam με πλήθος χαρακτηριστικών, όπως ο τύπος της ιδιοκτησίας, ο αριθμός των υπνοδωματίων, η γειτονιά κτλ. Στην συνέχεια εφαρμόστηκε καθαρισμός και προεπεξεργασία των δεδομένων για να τροφοδοτηθούν στα μοντέλα μηχανικής εκμάθησης. Πολλά μοντέλα μηχανικής μάθησης, συμπεριλαμβανομένου του δέντρου παλινδρόμησης, του τυχαίου δάσους και της μηχανής διανυσματικής υποστήριξης εκπαιδεύτηκαν και δοκιμάστηκαν στο σύνολο δεδομένων. Τα αποτελέσματα των διαφορετικών μοντέλων συγκρίθηκαν ως προς την ακρίβεια πρόβλεψής αξιοποιώντας ως μετρική την MSE και την R2. Τα αποτελέσματα έδειξαν ότι το μοντέλο XGBoost είχε την υψηλότερη προγνωστική ακρίβεια. Ακόμα, εξετάστηκε η σημαντικότητα των χαρακτηριστικών του συνόλου δεδομένων ως προς την συμβολή τους στην πραγματοποίηση ακριβέστερων προβλέψεων. Εδώ το σημαντικότερο χαρακτηριστικό αποδεικνύετε πως είναι το πλήθος των ατόμων που μπορούν να διαμείνουν σε ένα κατάλυμα. Συνολικά, τα αποτελέσματα αυτής της μελέτης καταδεικνύουν την αποτελεσματικότητα των μοντέλων μηχανικής εκμάθησης στην πρόβλεψη της τιμής μιας καταχώρισης Airbnb και υπογραμμίζουν τη σημασία της εξέτασης τόσο της ακρίβειας πρόβλεψης όσο και της επιρροής της από τα σημαντικότερα χαρακτηριστικά.