Εμπλουτίζοντας σχήματα ταξινόμησης ιατρικών εικόνων με ιδιότητες επεξηγησιμότητας
Enhancing medical imaging classification schemes with explainability properties
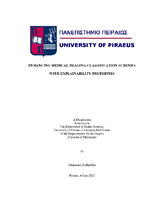
Doctoral Thesis
Συγγραφέας
Καλλιπολίτης, Αθανάσιος
Kallipolitis, Athanasios
Ημερομηνία
2023-06-06Επιβλέπων
Μαγκλογιάννης, ΗλίαςMaglogiannis, Ilias
Προβολή/ Άνοιγμα
Λέξεις κλειδιά
Explainability ; Interpretability ; Machine learning ; Medical imaging ; Image classification ; Artificial intelligence algorithms ; Deep learning ; Activation mapsΠερίληψη
Στην εκκίνηση ενός ταξιδιού αυτοβελτιώσης, ένας μηχανικός μηχανικής μάθησης ανησυχεί για την απόδοση του αλγόριθμου μηχανικής μάθησης που εκφράζεται ως επί το πλείστον με όρους σφάλματος μεροληψίας. Το πρώτο βήμα ωρίμανσης περιλαμβάνει την αναγνώριση της υποπροσαρμογής και της υπερπροσαρμογής ως μέρος μιας διαδικασίας μηχανικής μάθησης που εκμεταλλεύεται μόνο ένα μικρό σύνολο δεδομένων, ως αντιπροσωπευτικό μέρος της εκ των προτέρων γνώσης, προκειμένου να λυθεί ένα περίπλοκο και πολυπαραγοντικό πρόβλημα. Αναπόφευκτα, ένας «ώριμος» επαγγελματίας μηχανικής μάθησης πρέπει να κατανοήσει τη σημασία της γενίκευσης, της αντίστοιχης διακύμανσης και των μη αναγώγιμων σφαλμάτων, εάν πρόκειται να εξελιχθεί σε έναν «ώριμο» ειδικό μηχανικής μάθησης. Μαθαίνουμε να λογοδοτούμε για τη δουλειά μας μετρώντας την απόδοση μιας προτεινόμενης μεθοδολογίας. Είναι μια ισχυρή απαίτηση οι μετρήσεις αξιολόγησης όπως η ακρίβεια, η ισορροπημένη ακρίβεια, η ακρίβεια, η ανάκληση και άλλες σύμφωνα με τη φύση μιας εργασίας μετρικές μηχανικής μάθησης να διατηρούνται σε υψηλές τιμές, αλλά υπάρχουν εξίσου σημαντικές πτυχές που πρέπει να ληφθούν υπόψη. Η ανακάλυψη των κύριων και βοηθητικών αιτιών βάσει των οποίων ένα μοντέλο πρόβλεψης αποφασίζει για μια κλάση υπέρ μιας άλλης μπορεί να είναι μια ισχυρή πηγή χρήσιμης γνώσης και, ως εκ τούτου, πρέπει να πέσει φως στους εσωτερικούς μηχανισμούς λήψης αποφάσεων. Όπως είναι φυσικό, το ταξίδι της αυτοβελτίωσης είναι ένας ατελείωτος βρόχος, καθώς η συνειδητοποίηση της αποκτηθείσας γνώσης οδηγεί σε νέα αναπάντητα ερωτήματα.Για αυτή τη διατριβή, η ωρίμανση του συγγραφέα με τη χρήση βασικών εννοιών μηχανικής μάθησης έχει εξελιχθεί σε μια αναζήτηση για την ανάπτυξη προσεγγίσεων μηχανικής μάθησης για ταξινόμηση εικόνων που, εγγενώς ή εκ των υστέρων, έχουν την ικανότητα να παρέχουν ουσιαστικές συνδέσεις μεταξύ του προβλεπτικού αποτελέσματος και των οπτικών μοτίβων που το επηρέασαν περισσότερο. Δεδομένου ότι οι παραδοσιακές προσεγγίσεις μηχανικής μάθησης παραμένουν στο πεδίο ως αποτελεσματικές λύσεις για προβλήματα που καλύπτονται με λίγα δεδομένα, οι προτεινόμενες μεθοδολογίες καλύπτουν τόσο την παραδοσιακή μηχανική μάθηση όσο και τις αρχιτεκτονικές βαθιάς μάθησης. Το εύρος της διατριβής περιορίζεται στην ιατρική απεικόνιση και την εφαρμογή εξηγήσιμων προσεγγίσεων μηχανικής μάθησης για τον χειρισμό αντίστοιχων θεμάτων που σχετίζονται με την υγεία. Οι ιατρικές εικόνες θεωρούνται μια από τις πλουσιότερες πηγές πληροφοριών σχετικά με δεδομένα υγείας και τη βάση πάνω στην οποία οι ειδικοί λαμβάνουν αποφάσεις για θεραπευτικά σχήματα και παρεμβάσεις. Η δημιουργία εξηγήσιμων αυτοματοποιημένων συστημάτων που υποστηρίζουν αυτές τις αποφάσεις μπορεί να επιταχύνει τη διαδικασία ενσωμάτωσής τους στις καθημερινές κλινικές ροές εργασιών, καθώς οι ειδικοί θα μπορούν να κατανοούν και να εμπιστεύονται τις παραγώμενες προβλέψεις μέσω πρόσθετης διαφάνειας και αιτιότητας. Προς την ενσωμάτωση εξηγήσιμων ιδιοτήτων σε σχήματα ταξινόμησης μηχανικής μάθησης, σε αυτή τη διατριβή προτείνεται ένα νέο σχήμα εξήγησης που βασίζεται στο παράδειγμα Bag of Visual Words για την ερμηνεία των αποτελεσμάτων ταξινόμησης εικόνων μέσω συνόλου εξηγήσιμων ταξινομητών.Εφόσον τα Fisher Vectors ωθούν την απόδοση των προσεγγίσεων που βασίζονται στο λεξιλόγιο σε υψηλότερες τιμές, η προτεινόμενη μεθοδολογία εξελίσσεται με τη χρήση παραγωγικών μοντέλων, όπως τα Gaussian Mixture Models. Όσον αφορά τις τεχνικές βαθιάς μάθησης, προτείνεται μια νέα τμηματική προσέγγιση εξήγησης που εκμεταλλεύεται τα πλεονεκτήματα δύο καθιερωμένων προσεγγίσεων, των Χαρτών ενεργοποίησης κλάσεων βάσει παραγώγου και των Superpixels. Τα αποτελέσματα δείχνουν ότι το συνδυασμένο σχήμα αυξάνει σημαντικά την απόδοση της αρχικής προσέγγισης που βασίζεται σε κλίση και η αρθρωτή διάταξη επιτρέπει την εφαρμογή σε διαφορετικές προσεγγίσεις επεξήγησης.