Depression recognition from speech
Ανάλυση ηχητικών εγγραφών ή βίντεο για την αναγνώριση της κατάθλιψης
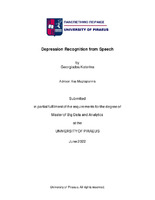
Master Thesis
Author
Georgiadou, Aikaterini
Γεωργιάδου, Αικατερίνη
Date
2022-06View/ Open
Keywords
Depression recognition ; Daic-Wox dataset ; Audio classification ; Machine learning ; Audio analysis ; Αναγνώριση κατάθλιψης ; Κατηγοριοποίηση ηχητικών δεδομένων ; Μηχανική μάθηση ; Ιατρικά δεδομέναAbstract
Depression, also known as major depressive disorder, is a major mental health disorder that is affecting ever more lives worldwide. It has a negative impact on the emotional, physical, and psychological state of a person. For a person to be diagnosed with depression, a series of tests must be performed while a series of symptoms must be present for at least 2 continuous weeks. Depression’s most common symptoms include feeling down or feeling worthless, lack of interest in daily activities, anxiety, irritability, and reduced appetite. However, depression is possible to cure, and early detection increases exponentially the possibility of controlling the condition.
The complexity of the depression recognition process poses challenges for clinicians regarding both the accuracy of the diagnosis and the timely treatment, given that the disease can be undiagnosed for many months or years, and the fact that delays in the recognition and the treatment can be vital on the life of the patient. To that end, machine learning has been introduced to the medical field to provide tools capable of enhancing the time needed as well as the accuracy and precision of the recognition process, while minimizing human interference.
For this purpose, this thesis studies the use of machine learning models for Depression Recognition using audio data from the widely known database DAIC-WOZ which contains clinical interviews designed specifically to support the diagnosis of psychological distress conditions. Regarding the audio information, the collaborative voice analysis repository (COVAREP) features provided by the dataset were used. Classification is performed using the following models Decision Tree, Random Forest, AdaBoost, Support Vector Machine, and Multilayer Perceptron. AdaBoost achieved the best results and is considered a good model for depression prediction.