Υλοποίηση και πραγματοποίηση αλγορίθμων προληπτικής συντήρησης
Design and implementation of predictive maintenance algorithms
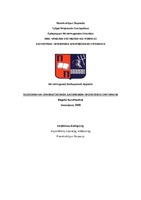
Προβολή/ Άνοιγμα
Λέξεις κλειδιά
Διορθωτική συντήρηση (Reactive maintenance) ; LSTM ; SVM ; Matrix profile ; Τεχνικές ανίχνευσης ακραίων τιμών ; Συντήρηση ; Μηχανική μάθηση ; One class support vector machine ; Προγνωστική συντήρηση (Proactive maintenance) ; Προβλεπτική συντήρηση (Predictive maintenance) ; Προληπτική συντήρηση (Preventive maintenance ή PMS) ; Gradient boosting ; Anomaly detection ; Είδη ανωμαλιών ; Ανίχνευση ανωμαλιών στη ναυτιλία ; Level anomaly detector ; Gradient boosting classification approach ; Weighted permutation entropy ; Second-level anomaly detector ; Single channel LSTMΠερίληψη
Δεδομένου ότι τα τελευταία χρόνια τα εμπορικά πλοία αποστέλλουν αυξανόμενα ποσά δεδομένων από τους εγκατεστημένους σε αυτά αισθητήρες στα κέντρα δεδομένων τους, έχει προκύψει η ανάγκη για βελτιωμένα εργαλεία ανάλυσης δεδομένων για να βοηθήσουν τους μηχανικούς, οι οποίοι παρακολουθούν τα δεδομένα αυτά, να μειώσουν τους κίνδυνους (λειτουργικούς, επιχειρησιακούς κτλ). Λαμβάνοντας υπόψιν ότι η Προγνωστική Συντήρηση αποτελεί πολύτιμο κομμάτι της μείωσης του συνολικού κόστους και του λειτουργικού κινδύνου, σε αυτό το δοκίμιο, επιδεικνύουμε την αποτελεσματικότητα ορισμένων προσεγγίσεων Anomaly Detection (Αναγνώρισης Ανωμαλιών) σε δεδομένα χρονοσειρών, που βασίζονται στη Μηχανική Μάθηση καθώς και στη Στατιστική Ανάλυση, όπως τα δίκτυα LSTM, το SVM One Class, το Matrix Profile, Έλεγχος συνοχής κλπ. Σκοπό των ανωτέρω είναι η πρόβλεψη της κατάστασης ορισμένων τμημάτων της κύριας μηχανής του πλοίου. Τα δεδομένα για τα οποία μελετήσαμε τις διαφορετικές προσεγγίσεις προήλθαν από ροές δεδομένων αισθητήρων εγκατεστημένων σε 10 σκάφη μιας Μεγάλης Ελληνικής Ναυτιλιακής Εταιρείας. Τέλος, έχοντας λάβει υπόψιν τα αποτελέσματα των δοκιμών αυτών προτείνουμε ένα συμπληρωματικό μοντέλο που αποτελείται από ένα συνδυασμό των προσεγγίσεων που μελετήθηκαν υποσχόμενοι καλύτερα αποτελέσματα. Το προτεινόμενο συνδυαστικό μοντέλο αποτελείται από δύο επίπεδα, σαν πρώτο βήμα εκτελείται μια πρόβλεψη να αυξήσει έναν συναγερμό για υψηλό δυναμικό μιας ανωμαλίας στα εισερχόμενα δεδομένα, ενώ το δεύτερο δίνει μια πιθανότητα αυτής της ανωμαλίας να υποδεικνύει το ελάττωμα που μελετήσαμε σχετικά με το Crosshead Bearing.