Δημιουργία γνώσης βάσει δεδομένων μεγάλης κλίμακας για την γνωσιακή διαχείριση τηλεπικοινωνιακών υποδομών
Knowledge generation from telecommunications big data for enabling cognitive infrastructure management
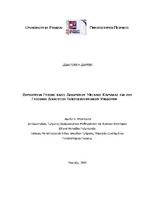
Προβολή/ Άνοιγμα
Λέξεις κλειδιά
Συστήματα γνωσιακής διαχείρισης ; Δημιουργία γνώσης ; Μηχανική μάθηση ; Δεδομένα μεγάλης κλίμακας ; Μη καθοδηγούμενες τεχνικές μάθησης ; Cognitive Radio Systems (CRSs) ; Cognitive learning ; Knowledge building ; Machine learning ; Big data ; Unsupervised learningΠερίληψη
Η συνεχώς αυξανόμενη χρήση του Διαδικτύου και η βελτιστοποίηση των υπηρεσιών, υπό την έννοια της προσφοράς περισσότερων δυνατοτήτων στους χρήστες, έχει ως αποτέλεσμα την αυξανόμενη ανάγκη ραδιοσυχνοτήτων, μίας περιορισμένης φυσικής πηγής, και των επεξεργαστικών δυνατοτήτων των δικτύων. Τα συστήματα γνωσιακής διαχείρισης έχουν την ικανότητα να βελτιώνουν την κατανομή και την χρησιμοποίηση των πόρων ενώ παράλληλα διατηρούν, αν όχι βελτιώνουν, την ποιότητα των υπηρεσιών (QoS) και την ποιοτικά της εμπειρίας των χρηστών.
Παράλληλα, η μεγάλη διαθεσιμότητα της αδόμητης πληροφορίας από διαφορετικές πηγές παρέχει την δυνατότητα της δημιουργίας γνώσης αλλά η μεγάλη ταχύτητα με την οποία η πληροφορία αυτή αλλάζει απαιτεί τέτοια τεχνολογία που να μπορεί να επεξεργάζεται μεγάλο όγκο δεδομένων σε μικρά χρονικά διαστήματα. Τα δεδομένα που χαρακτηρίζονται από μεγάλο όγκο, ποικιλομορφία και ταχύτητα είναι γνωστά ως Big
Data.
Οι μηχανισμοί δημιουργίας γνώσης αναφέρονται συχνά ως η διέξοδος και στις 2 παραπάνω προκλήσεις των μελλοντικών δικτύων. Συγκεκριμένα, οι μηχανισμοί δημιουργίας γνώσης παράγουν δυναμικά την γνώση που περιλαμβάνει την πρότερη εμπειρία του δικτύου και μπορεί να καθοδηγήσει τις αποφάσεις του δικτύου. Συγκεκριμένα, παρακολουθούν την κατάσταση του δικτύου, συλλέγουν πληροφορίες από το περιβάλλον τους και σχετικά με την απόδοση των αποφάσεων τους και εκπαιδεύονται δυναμικά ώστε να επιλέγουν την καταλληλότερη των αποφάσεων δεδομένης της κατάστασης του δικτύου. Κατά την διαδικασία λήψης αποφάσεων για το δίκτυο, οι κανόνες και η πολιτική διαχείρισης του δικτύου συνυπολογίζονται. Προς αυτήν την κατεύθυνση, η εν λόγω διατριβή μελετά, σχεδιάζει, προτείνει και αξιολογεί μηχανισμούς δημιουργίας γνώσης που μπορούν να αξιοποιήσουν δεδομένα μεγάλης κλίμακας και να βελτιώσει τις διαδικασίες λήψης αποφάσεων των συστημάτων γνωσιακής διαχείρισης.