Small-object detection in remote sensing images and video
Ανίχνευση μικρών αντικειμένων σε εικόνες και βίντεο τηλεπισκόπησης
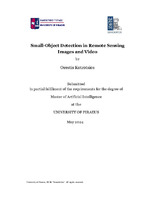
Master Thesis
Συγγραφέας
Kotrotsios, Orestis
Κοτρώτσιος, Ορέστης
Ημερομηνία
2024-05Επιβλέπων
Maglogiannis, IliasΜαγκλογιάννης, Ηλίας
Προβολή/ Άνοιγμα
Λέξεις κλειδιά
Remote sensing images ; Object detection ; Deep learning ; Machine learningΠερίληψη
Η ανίχνευση αντικειμένων σε εικόνες τηλεπισκόπησης αποτελεί ένα δύσκολο πρόβλημα για την ερευνητική κοινότητα της υπολογιστικής όρασης, επειδή τα αντικείμενα σε τέτοιες εικόνες έχουν πολύ λίγα εικονοστοιχεία (10-20 εικονοστοιχεία). Έχουν υπάρξει πολλές βελτιώσεις στο mean Average Precision (mAP) των μοντέλων με τη χρήση διαφόρων τεχνικών, αλλά όλες αυτές οι βελτιώσεις έχουν κάποιο κόστος. Τα μοντέλα ανίχνευσης γίνονται όλο και μεγαλύτερα, κάτι που μπορεί να προκαλέσει πρόβλημα ειδικά όταν κάποιος θέλει να εφαρμόσει ένα μοντέλο ανίχνευσης σε ένα δορυφόρο ή ένα μη επανδρωμένο εναέριο όχημα, καθώς οι υπολογιστικές τους δυνατότητες είναι περιορισμένες.
Η παρούσα διατριβή προτείνει έναν ευέλικτο gradient-path design σε επίπεδο δικτύου που μπορεί να εφαρμοστεί τόσο σε μοντέλα ενός σταδίου όσο και σε μοντέλα πολλαπλών σταδίων με αρχιτεκτονική παρόμοια με το “PaNet”. Η μέθοδος αυτή μειώνει τις υπολογιστικές απαιτήσεις του μοντέλου χρησιμοποιώντας μόνο το μισό του feature map από το “backbone” στο στάδιο του “neck”, ενώ το άλλο μισό παρακάμπτει εντελώς το στάδιο του “neck”. Με αυτόν τον τρόπο δημιουργούμε ένα gradient path που συνδέει απευθείας τα prediction heads με τα layers του “backbone”, ελαχιστοποιώντας έτσι την απώλεια πληροφοριών λόγω των μακρών gradient paths.
Η προτεινόμενη μεθοδολογία δοκιμάστηκε στο μοντέλο δύο σταδίων "PaNet" και στο μοντέλο ενός σταδίου "TPH-YOLOv5". Τα σύνολα δεδομένων που χρησιμοποιήθηκαν για την αξιολόγηση των μοντέλων με την προτεινόμενη μέθοδο, ήταν τα Microsoft Common Object in COntext (MS COCO), VisDrone και Aerial Image Tiny Object detection (AI-TOD).
Η προτεινόμενη μέθοδος πέτυχε μείωση των GFLOPs (Giga Floating Point Operations Per Second) στο “PaNet” κατά 9,51%, ενώ στο “TPH-YOLOv5” η μείωση ήταν 32,67%.
Ταυτόχρονα, η μέση μέση ακρίβεια του “PaNet” με την προτεινόμενη μέθοδο μειώθηκε επίσης κατά 5,7% όταν το κατώτερο όριο του Intersection over Union (IoU) ήταν 50% και κατά 3,2% στο μέσο mAP για IoU με κατώτερο όριο από 50% έως 95%, στο σύνολο δεδομένων MS COCO. Επιπλέον, το mAP του “PaNet” με την προτεινόμενη μέθοδο μειώθηκε κατά 7,8% και η μέση mAP κατά 3,6% στο σύνολο δεδομένων AI-TOD, σε σχέση με τις επιδώσεις του “PaNet” χωρίς την προτεινόμενη μέθοδο.
Ωστόσο, το “TPH-YOLOv5” με την προτεινόμενη μέθοδο μείωση μόνο 1,6% τόσο στο mAP όσο και στο μέσο mAP στο σύνολο δεδομένων του VisDrone. Επιπλέον, στο σύνολο δεδομένων AI-TOD η προτεινόμενη μέθοδος είχε καλύτερη απόδοση από την πρωτότυπη κατά 6,4% mAP και κατά 2,4% στο μέσο mAP.