Ανάλυση δεδομένων καλαθοσφαίρισης για την πρόβλεψη αποτελεσμάτων με επιλογή χαρακτηριστικών
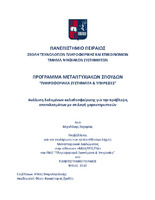
Προβολή/ Άνοιγμα
Λέξεις κλειδιά
Basketball ; Euroleague ; Sports Analytics ; Machine learning ; Logistic regression ; Support vector machine ; Random forest ; k-nearest neighbors ; Big dataΠερίληψη
Η παρούσα Μεταπτυχιακή Διπλωματική εργασία εμβαθύνει στον τομέα της Ανάλυσης Αθλητικών Δεδομένων (Sports Analytics) από το άθλημα της Καλαθοσφαίρισης (Basketball) με σκοπό την πρόβλεψη αποτελεσμάτων και την επιλογή χαρακτηριστικών (Feature Selection) που συμβάλλουν στην επίτευξη αυτού του στόχου. Η εργασία αναπτύσσει και εφαρμόζει σύγχρονες μεθοδολογίες μηχανικής μάθησης (Machine Learning) και στατιστικής ανάλυσης για την επεξεργασία και την αξιολόγηση των δεδομένων, επιδιώκοντας να αναγνωρίσει ποια δεδομένα και στατιστικά στοιχεία έχουν την μεγαλύτερη προβλεπτική αξία.
Η έρευνα αρχίζει με μια εκτενή ανασκόπηση της σχετικής βιβλιογραφίας, εξετάζοντας προηγούμενες μελέτες που έχουν ασχοληθεί με την πρόβλεψη αποτελεσμάτων στον αθλητισμό και ειδικότερα στην καλαθοσφαίριση. Στη συνέχεια, παρουσιάζεται η μεθοδολογία που υιοθετήθηκε για την επεξεργασία των δεδομένων, η οποία περιλαμβάνει τεχνικές προεπεξεργασίας, επιλογής χαρακτηριστικών, και μοντελοποίησης. Ειδική έμφαση δίνεται στην εφαρμογή αλγορίθμων μηχανικής μάθησης, όπως η Logistic Regression, k-nearest neighbors (KNN), Support Vector Machine (SVM), Random Forest, για την αξιολόγηση της προβλεπτικής τους ικανότητας σε σχέση με τα αποτελέσματα των αγώνων.
Ακολούθως, παρουσιάζονται τα αποτελέσματα της εφαρμογής των μεθόδων αυτών σε ένα σύνολο δεδομένων που περιλαμβάνει στατιστικά από επαγγελματικούς αγώνες καλαθοσφαίρισης και συγκεκριμένα στο Ευρωπαϊκό Πρωτάθλημα (Euroleague). Η ανάλυση επικεντρώνεται στην αξιολόγηση της σημασίας κάθε χαρακτηριστικού και της συνεισφοράς του στην πρόβλεψη του αποτελέσματος των αγώνων, καθώς και στην αποτελεσματικότητα των διαφορετικών μοντέλων πρόβλεψης. Απώτερος στόχος ήταν να κάνουμε πρόβλεψη αποτελέσματος με τους παραπάνω κατηγοριοποιητές βάσει του διαθέσιμου συνόλου δεδομένων.
Τέλος, η εργασία καταλήγει με μια συζήτηση για τις προκλήσεις και τις προοπτικές της πρόβλεψης αποτελεσμάτων στην καλαθοσφαίριση, προτείνοντας διευρύνσεις για μελλοντικές ερευνητικές προσπάθειες. Επιπλέον, αναγνωρίζεται η σημασία της ενσωμάτωσης περισσότερων δεδομένων και της βελτίωσης των αλγορίθμων μηχανικής μάθησης για την αύξηση της ακρίβειας των προβλέψεων. Η εργασία αυτή συμβάλλει στον τομέα της αναλυτικής αθλητικής επιστήμης, προσφέροντας πολύτιμες διορατικότητες για την εφαρμογή της μηχανικής μάθησης στην πρόβλεψη αθλητικών αποτελεσμάτων.