Management of cellular broadband networks by means of machine learning techniques
Διαχείριση ετερογενών ευρυζωνικών δικτύων με χρήση μηχανισμών μηχανικής μάθησης
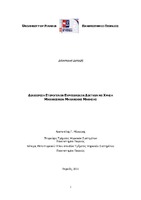
Doctoral Thesis
Συγγραφέας
Margaris, Aristotelis
Μάργαρης, Αριστοτέλης Γ.
Ημερομηνία
2021-02-05Προβολή/ Άνοιγμα
Λέξεις κλειδιά
3GPP ; Het-Net ; Ultra-Dense Networks ; Optimization ; Network management ; Knowledge-building ; High-Level Objectives ; Energy efficiency ; EMF reduction ; Quality of service ; Fault prevention ; Network KPI Forecasting ; Network element clustering ; Self-organizing maps ; Growing neural gas ; Neural networks ; Gradient boosted treesΠερίληψη
Τα κυψελωτά δίκτυα κινητών επικοινωνιών είναι μία από τις τεχνολογίες με την μεγαλύτερη επίδραση στην σημερινή βιομηχανία των τεχνολογιών επικοινωνιών και πληροφορικής. Παρέχουν ασύρματη πρόσβαση στο διαδίκτυο αλλά και μια πληθώρα άλλων υπηρεσιών με πάρα πολύ υψηλή διαθεσιμότητα και αποτελεσματικότητα. Η εξέλιξη αυτής της τεχνολογίας έρχεται με την ωρίμανση των δικτύων προδιαγραφών 3GPP, η πρόοδος των οποίων υπόσχεται να παρέχει ακόμα υψηλότερη ποιότητα υπηρεσιών, περισσότερες δυνατότητες αλλά και λύσεις σε προβλήματα των παλαιότερων γενεών. Για την επίτευξη αυτών των στόχων, οι κατασκευαστές αυτής της τεχνολογίας πρέπει να αναλύσουν προσεκτικά την πολυπλοκότητα αυτών των δικτύων αλλά και των δυνατοτήτων εγκατάστασης τους και να παρέχουν ευφυές λογισμικό διαχείρισης τους. Διαφοροποιήσεις σε κυψελωτά δίκτυα τύπου 3GPP όπως τα ετερογενή κυψελωτά δίκτυα αλλά και τα «υπερ-πυκνά» δίκτυα είναι εξελίξεις αυτών των δικτύων με αυξημένη πολυπλοκότητα και δυνατότητες που βρίσκεται στη βιβλιογραφία. Μέσω αυτών των δυνατοτήτων μπορούμε να βελτιώσουμε τους διάφορους λειτουργικούς στόχους της υποδομής όπως ενεργειακή αποδοτικότητα, δικτυακές επιδόσεις και αποφυγή σφαλμάτων. Αυτή η διδακτορική διατριβή έχει ως σκοπό να αναλύσει διαφορετικές προσεγγίσεις βελτιστοποίησης ετερογενών δικτύων καταλήγοντας έτσι σε προτάσεις για επέκταση τους επηρεάζοντας όσο το δυνατών περισσότερους στόχους-κλειδιά. Στατική διαχείριση και ρύθμιση σε συνδυασμό με συλλογή γνώσης θα χρησιμοποιηθεί για την βελτίωση ενεργειακή επίδοσης σεναρίων-κλειδιών για την 4η γενιάς κινητής τηλεφωνίας. Αλγόριθμοι δυναμικού διαμοιρασμού πόρων θα χρησιμοποιηθούν σαν μία μέθοδος διαχείρισης πραγματικού χρόνου με σκοπό την βελτίωση ποιότητας υπηρεσιών σε μικρό-κλίμακα. Τέλος, μοντέλα μηχανικής μάθησης θα εκπαιδευτούν σε ιστορικά δεδομένα με σκοπό την πρόβλεψη των λειτουργικών δεικτών του δικτύου, εκτίμηση της πιθανότητας δικτυακής υπερφόρτωσης και αναγνώριση άγνωστων ομάδων δικτυακών στοιχείων βασισμένα στην συμπεριφορά τους. Αυτές οι προβλέψεις θα βοηθήσουν την διαχείριση της υποδομής στην ενεργοποίηση αντίμετρων για την αποφυγή της υποβάθμισης της ποιότητας υπηρεσιών αλλά και την επικράτηση των προδιαγραφών της τεχνολογίας. Αυτές θα οδηγήσουν επίσης στην ελάττωση του OPEX αλλά και του ενεργειακού αποτυπώματος του συστήματος οδηγώντας έτσι σε βιώσιμες και επιτυχημένες επενδύσεις για τους παρόχους. Το πλαίσιο ανάπτυξης για αυτούς τους αλγορίθμους είναι ένα αυτοσχέδιο πρωτότυπο προσομοιωτή ετερογενών δικτύων και πλατφόρμα εκτέλεσης πειραματικών αλγορίθμων. Αυτό το σύστημα σχεδιάστηκε με βάση τις πρότυπες προδιαγραφές προσομοίωσης τέτοιων συστημάτων και τα ρεαλιστικά δεδομένα που θα εξαχθούν από αυτό θα βοηθήσουν στην υπεράσπιση της αποτελεσματικότητας των προτεινόμενων αλγορίθμων για την ένταξη τους στην τεχνολογία κυψελωτών επικοινωνιών 3GPP.