Anomaly detection for industrial control systems
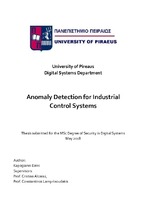
Master Thesis
Συγγραφέας
Καπογιάννη, Ειρήνη
Ημερομηνία
2018Προβολή/ Άνοιγμα
Λέξεις κλειδιά
Anomaly detection ; Machine learning ; Industrial control systems ; Machine learning algorithmsΠερίληψη
As Industrial Control Systems (ICSs) become more and more connected it follows that they need to
become more secure. Traditional Intrusion Detection Systems (IDSs) do not work well due to the fact
that they mostly work on a signature basis and there are not many known signatures to detect attacks
on ICSs. Since the network traffic from an ICS is claimed to be static and signatures are scarce, searching
for anomalies in the network to detect threats is more effective. This can be achieved using machine
learning and other statistical models, teaching the system to tell regular traffic from irregularities. In
this thesis we survey different anomaly detection techniques, which based on different parameters, we
evaluate and point the one that can fit better. Based on the survey and the risk analysis we analyze the
algorithm on which we conclude, and with real-time data-sets (normal and anomalous) we do an
implementation. From this work we propose and evaluate methods to be used when creating a more
data driven IDS, capable of detecting process semantic tampering within an ICS. Our results from
conducted experiments exhibit a static nature of the data originating from the ICS and the result from
evaluating many different proposed anomaly detections using proof of concept systems, we deem that
the anomaly detection and algorithm that we conclude works well for both semantic tampering as well
as on a network basis. Having an IDS using a fusion this proposed method, would benefit the security of
an ICS.