Value based and network Data Envelopment Analysis : new models and applications
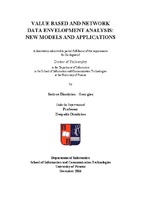
Doctoral Thesis
Συγγραφέας
Σωτήρος, Δημήτριος - Γεώργιος Π.
Sotiros, Dimitrios - Georgios
Ημερομηνία
2016-12Επιβλέπων
Δεσπότης, ΔημήτριοςΠροβολή/ Άνοιγμα
Λέξεις κλειδιά
Data Envelopment Analysis (DEA) ; Value judgments ; Value based DEA ; Max column normalization ; Network DEA ; Composition paradigm in network DEA ; Academic research activity ; Higher Education ; Περιβάλλουσα ανάλυση δεδομένων ; Περιβάλλουσα ανάλυση αξιών ; Κανονικοποίηση των δεδομένων ; Πολυσταδιακή περιβάλλουσα ανάλυση δεδομένων ; Ερευνητική δραστηριότητα ακαδημαϊκών ; Ανώτατη ΕκπαίδευσηΠερίληψη
Η μέτρηση της αποδοτικότητας των συστημάτων παραγωγής αποτελεί καθοριστικό παράγοντα για την βελτίωση τους. Η αποτίμηση της αποδοτικότητας επιτυγχάνεται με τη χρήση παραμετρικών ή μη-παραμετρικών τεχνικών. Η Περιβάλλουσα Ανάλυση Δεδομένων - ΠΑΔ (Data Envelopment Analysis - DEA) αποτελεί μια από τις δημοφιλέστερες μη-παραμετρικές τεχνικές για την εκτίμηση της αποδοτικότητας ομοειδών μονάδων ενός συστήματος, επί τη βάσει πολλαπλών εισροών και εκροών και στηρίζεται στο γραμμικό προγραμματισμό. Σύγχρονες επεκτάσεις της ΠΑΔ αποτελούν η Περιβάλλουσα Ανάλυση Αξιών (value based DEA) και η πολυσταδιακή Περιβάλλουσα Ανάλυση Δεδομένων (network DEA). Η Περιβάλλουσα Ανάλυση Αξιών στηρίζεται σε τεχνικές της Πολυκριτηριακής Ανάλυσης Αποφάσεων (Multiple Criteria Decision Analysis - MCDA) για το μετασχηματισμό των επιπέδων των εισροών και εκροών σε αξίες. Ο μετασχηματισμός αυτός εξυπηρετεί στην ενσωμάτωση των προτιμήσεων του εκάστοτε αναλυτή, σχετικά με τις συναρτήσεις αξιών των εισροών/εκροών, στην εκτίμηση της αποδοτικότητας των μονάδων. Παρόλο που τα μοντέλα της Περιβάλλουσας Ανάλυσης Αξιών που προτείνονται στη βιβλιογραφία παρέχουν ευκολία στην εισαγωγή προτιμήσεων σχετικά με τις συναρτήσεις αξιών των εισροών/εκροών, διαχωρίζουν μόνο τις αποδοτικές από τις μη-αποδοτικές μονάδες και αδυνατούν να εκτιμήσουν την αποδοτικότητα κάθε αποτιμώμενης μονάδας. Η πολυσταδιακή Περιβάλλουσα Ανάλυση Δεδομένων αποτελεί μια από τις σημαντικότερες επεκτάσεις της κλασσικής Περιβάλλουσας Ανάλυσης Δεδομένων. Τα συμβατικά μοντέλα της ΠΑΔ θεωρούν ότι ο μηχανισμός παραγωγής συνίσταται σε ένα στάδιο. Παρόλα αυτά, υπάρχουν περιπτώσεις όπου ο αναλυτής γνωρίζει ότι ο μηχανισμός παραγωγής αποτελείται από υποδιαδικασίες (υποστάδια). Η πληροφορία αυτή επηρεάζει την εκτίμηση αποδοτικότητας και τα κλασσικά μοντέλα της ΠΑΔ αδυνατούν να αφομοιώσουν την πληροφορία αυτή. Η πολυσταδιακή Περιβάλλουσα Ανάλυση Δεδομένων αντιλαμβάνεται το μηχανισμό παραγωγής ως ένα δίκτυο από υποστάδια, τα οποία συνδέονται μεταξύ τους με ενδιάμεσους παράγοντες (intermediate measures). Όμως, τα μοντέλα της Πολυσταδιακής Περιβάλλουσας Ανάλυσης Δεδομένων που παρουσιάζονται στη βιβλιογραφία, δεν προσδιορίζουν ένα μοναδικό δείκτη αποδοτικότητας στα επιμέρους στάδια (υποστάδια) για κάθε αποτιμώμενη μονάδα. Επίσης, η εκτίμηση της αποδοτικότητας επιτυγχάνεται εισάγοντας άγνωστη στάθμιση στα υποστάδια αυτά. Τα ζητήματα αυτά θέτουν σε αμφισβήτηση την εγκυρότητα των αποτελεσμάτων καθώς, για κάθε αποτιμώμενη μονάδα, δίνεται διαφορετική και άγνωστη στάθμιση στα επιμέρους στάδια για τη διαμόρφωση του δείκτη αποδοτικότητας. Στο πρώτο μέρος της παρούσας διδακτορικής διατριβής, επικεντρωνόμαστε στην Περιβάλλουσα Ανάλυση Αξιών. Στόχος είναι η ανάπτυξη ενός νέου μοντέλου το οποίο, σε αντίθεση με τα μοντέλα της Περιβάλλουσας Ανάλυσης Αξιών που προτείνονται στη βιβλιογραφία, εκτιμά την αποδοτικότητα κάθε αποτιμώμενης μονάδας. Συγκεκριμένα, εισάγουμε ένα μετασχηματισμό των δεδομένων και των μεταβλητών των γραμμικών μοντέλων της ΠΑΔ, με τον οποίο οι νέες μεταβλητές εκφράζουν πλέον αξία αντί για βάρη. Δείχνουμε ότι ο μετασχηματισμός αυτός ενισχύει τα κλασσικά μοντέλα της ΠΑΔ με επιπλέον ιδιότητες και επίσης, ότι επιλύει το θέμα ασυνέχειας που παρουσιάζουν οι συναρτήσεις αξίας στην ΠΑΔ με μη γραμμικές εικονικές εισροές/εκροές. Τα ευρήματα αυτά, μας οδηγούν στη δημιουργία ενός νέου μοντέλου της Περιβάλλουσας Ανάλυσης Αξιών, το οποίο εκτιμά την αποδοτικότητα κάθε αποτιμώμενης μονάδας. Επίσης, εισάγουμε μια νέα μεθοδολογία για την εισαγωγή προτιμήσεων στα πλαίσια της ΠΑΔ μέσω Μονότονης Παλινδρόμησης (Ordinal Regression). Η αποτελεσματικότητα του νέου μοντέλου της Περιβάλλουσας Ανάλυσης Αξιών που αναπτύξαμε αναδεικνύεται μέσω της εφαρμογής του σε μια μελέτη περίπτωσης που έχει ήδη παρουσιασθεί στη βιβλιογραφία, καθώς και μέσα από την ανάπτυξη μιας νέας εφαρμογής που σχετίζεται με την αξιολόγηση της ερευνητικής δραστηριότητας ακαδημαϊκών καθηγητών, η οποία λαμβάνει υπόψη της την ποιοτική και ποσοτική διάσταση των δημοσιεύσεων των καθηγητών. Στο δεύτερο μέρος της παρούσας διδακτορικής διατριβής, επικεντρωνόμαστε στην πολυσταδιακή Περιβάλλουσα Ανάλυση Δεδομένων, όπου και αναπτύσσουμε μια νέα μεθοδολογία με στόχο την επίλυση των μειονεκτημάτων που χαρακτηρίζουν τα μοντέλα της πολυσταδιακής Περιβάλλουσας Ανάλυσης Δεδομένων που παρουσιάζονται στη βιβλιογραφία. Συγκεκριμένα, εισάγουμε μια προσέγγιση πολυκριτηριακού προγραμματισμού η οποία χρησιμοποιεί την L∞ μετρική, ώστε να υπολογίσει τις αποδοτικότητες των μονάδων στα υποστάδια όσο πιο κοντά γίνεται στα ιδανικά τους επίπεδα. Η προσέγγιση αυτή, σε αντίθεση με τα υπάρχοντα μοντέλα που προτείνονται στη βιβλιογραφία, παρέχει μοναδικό δείκτη αποδοτικότητας για κάθε μονάδα σε κάθε υποστάδιο και επίσης διαχειρίζεται τα επιμέρους υποστάδια με την ίδια βαρύτητα. Τα πλεονεκτήματα της νέας αυτής μεθοδολογίας γίνονται ιδιαίτερα σαφή όταν συγκρίνουμε τα αποτελέσματα της μεθοδολογίας που αναπτύξαμε με τα αποτελέσματα άλλων μοντέλων, που παρουσιάζονται στη βιβλιογραφία, πάνω σε συνθετικά δεδομένα και σε δεδομένα που έχουν ήδη μελετηθεί στη βιβλιογραφία. Η πρακτικότητα της παραπάνω μεθόδου αναδεικνύεται περαιτέρω μέσω της εφαρμογής της σε μια καινοτόμο μελέτη περίπτωσης, που σχετίζεται με την ερευνητική δραστηριότητα ακαδημαϊκών καθηγητών αναλυόμενη σε δύο στάδια συνδεδεμένα σειριακά.