Ζητήματα ομοιότητας στην εξόρυξη γνώσης: μεθοδολογίες και τεχνικές
Similarity issues in data mining: methodologies and techniques
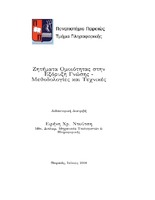
Προβολή/ Άνοιγμα
Θεματική επικεφαλίδα
Δίκτυα υπολογιστών ; Data mining ; Computer networks ; Εξόρυξη δεδομένων ; Online social networks ; Κοινωνικά δίκτυαΠερίληψη
Στις μέρες μας εξάγονται όλο και περισσότερα πρότυπα εξαιτίας της πληθώρας των δεδομένων και της ευρείας χρήσης της Διαδικασίας Ανακάλυψης Γνώσης από τα Δεδομένα και της Εξόρυξης Γνώσης. Αυτή η πληθώρα των προτύπων επιβάλει την αντιμετώπιση προβλημάτων που σχετίζονται με τη διαχείρισή τους. Μία από τις πιο σημαντικές λειτουργίες στα πρότυπα είναι αυτή της αποτίμησης της ομοιότητας μεταξύ προτύπων, ένα πρόβλημα που έχει πολλές εφαρμογές και εγείρει σημαντικά ερευνητικά θέματα. Στα πλαίσια της παρούσας διατριβής μελετάμε διάφορα θέματα που προκύπτουν κατά την αποτίμηση της ομοιότητας μεταξύ προτύπων. Πιο συγκεκριμένα, προτείνουμε αρχικά ένα πλαίσιο για την αποτίμηση της ομοιότητας μεταξύ προτύπων αυθαίρετης πολυπλοκότητας τα οποία ορίζονται τόσο πάνω σε πρωτογενή δεδομένα όσο και πάνω σε άλλα πρότυπα. Στη συνέχεια μελετάμε προβλήματα ομοιότητας για μερικούς από τους πιο δημοφιλείς τύπους προτύπων, συγκεκριμένα για συχνά στοιχειοσύνολα, δέντρα απόφασης και συστάδες. Ειδικότερα για την περίπτωση των συχνών στοιχειοσυνόλων, εξετάζουμε κατά πόσο οι παράμετροι της Εξόρυξης Γνώσης επηρεάζουν το αποτέλεσμα της σύγκρισης μεταξύ συνόλων από στοιχειοσύνολα. Στην περίπτωση των δέντρων απόφασης, προτείνουμε ένα πλαίσιο που βασιζόμενο στα δέντρα απόφασης αποτιμά την ομοιότητα τόσο μεταξύ δέντρων απόφασης όσο και μεταξύ συνόλων δεδομένων κατηγοριοποίησης. Τέλος, στην περίπτωση των συστάδων προτείνουμε μέτρα απόστασης μεταξύ συστάδων και συσταδοποιήσεων, τα οποία και χρησιμοποιούμε στη συνέχεια για να παρακολουθήσουμε την εξέλιξη και να εντοπίσουμε τυχόν αλλαγές σε δυναμικούς πληθυσμούς. Κοινό στοιχείο σε όλες τις παραπάνω περιπτώσεις είναι η θεώρηση ότι τα πρότυπα αποτελούνται από μία δομική και μία ποσοτική συνιστώσα, γεγονός που ανοίγει νέους δρόμους προς την κατεύθυνση ενός ενιαίου μοντέλου για τα αποτελέσματα της Εξόρυξης Γνώσης.