Clustering algorithm recommendation platform for distributed environments
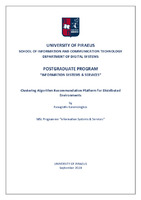
Master Thesis
Author
Karamolegkos, Panagiotis
Καραμολέγκος, Παναγιώτης
Date
2024-09View/ Open
Keywords
EverCluster ; Python ; Spark ; Machine learning ; Platform ; Artificial intelligence ; Docker ; Computing clusters ; Distributed computing ; Synthetic dataAbstract
The rapid growth of data in various fields has necessitated the development of advanced tools for effective data analysis. Clustering, a fundamental technique in machine learning, plays a crucial role in organizing and interpreting large datasets. However, selecting the most suitable clustering algorithm for specific data characteristics poses significant challenges, as it requires balancing computational speed and accuracy while considering the intricacies of dataset properties and algorithm parameters. This document presents EverCluster, a comprehensive, cloud-centric platform designed to streamline the clustering process. EverCluster automates the recommendation of optimal clustering algorithms by leveraging machine learning models that adapt to user preferences and dataset features. The architecture of EverCluster is detailed, featuring both high- and low-level descriptions supported by deployment and activity diagrams. Experimental findings highlight the platform's effectiveness, revealing a success rate of 65.5% for speed-based recommendations and an average of 81.1% for accuracy-based recommendations. The platform performs particularly well on datasets with fewer features and higher iteration numbers making the speed-based recommendations to reach success rates of 83.3%. By addressing the complexities involved in clustering algorithm selection and deployment, EverCluster aims to provide a valuable resource for data scientists and researchers, facilitating more efficient and accurate data analysis across diverse applications.