Incorporation of physical laws into neural networks for solving elasticity problems
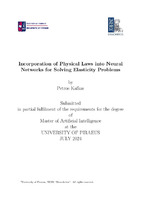
Master Thesis
Συγγραφέας
Kafkas, Petros
Καυκάς, Πέτρος
Ημερομηνία
2024-07Προβολή/ Άνοιγμα
Λέξεις κλειδιά
Physics informed machine learning ; Physics informed neural networks ; Elasto-static plate bending ; Boundary conditions ; Mindlin plate model ; Kirchhoff plate model ; Finite Element Analysis (FEA) ; Boundary condition enforcement ; Fourier feature embeddings ; Self-scalable hyperbolic tangent ; Training stability ; Partial Differential Equations (PDEs) ; Elasticity problems ; Isotropic plates ; Neural networks ; Simulation of plate bending ; Comparative analysis (PINNs vs. FEA)Περίληψη
This study investigates the performance of Physics-Informed-Neural-Networks (PINNs) in addressing elasto-static plate bending problems under various boundary conditions. To that effect, the bending of a square isotropic plate was simulated using the Mindlin and Kirchhoff models. The accuracy of the predictions is compared to the established method of Finite Element Analysis (FEA). For ensuring boundary condition compliance, a hard- enforced boundary method is adopted from the literature. Additionally, Fourier Feature Embeddings and Self-Scalable hyperbolic-tangent are employed for increased training stability. The findings confirm the results from previous studies regarding the ability of PINNs to successfully tackle electrostatic problems and confirm that PINNs show great promise as a novel method for solving Partial Differential Equations (PDEs).