Τεχνικές data mining και προβλεπτική αναλυτικής σακχαρώδους διαβήτη
Data mining techniques and predictive analysis for diabetes mellitus
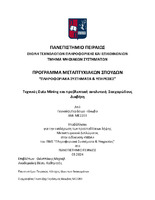
Προβολή/ Άνοιγμα
Λέξεις κλειδιά
Σακχαρώδης διαβήτης ; Μηχανική μάθηση ; Λογιστική παλινδρόμηση ; Τυχαίο δάσος ; K-πλησιέστερων γειτόνων ; Κανονικοποίηση ; Τεχνική κλιμάκωσης ; Μηχανική χαρακτηριστικώνΠερίληψη
Ο σακχαρώδης διαβήτης είναι μια διαδεδομένη χρόνια νόσος με σημαντικές επιπτώσεις στη δημόσια υγεία και την ατομική ευημερία. Τα τελευταία χρόνια, οι τεχνικές μηχανικής μάθησης (MM) έχουν αναδειχθεί ως ισχυρά εργαλεία για την πρόβλεψη των αποτελεσμάτων του διαβήτη, προσφέροντας τη δυνατότητα βελτίωσης της έγκαιρης ανίχνευσης. Αυτή η εργασία παρουσιάζει μια ολοκληρωμένη αξιολόγηση διαφόρων μοντέλων ΜΜ, συμπεριλαμβανομένης της λογιστικής παλινδρόμησης (LR), των μηχανών διανυσμάτων υποστήριξης (SVM), του τυχαίου δάσους (RF), των k-πλησιέστερων γειτόνων (KNN), των πολυστρωματικών perceptrons (MLPs) και του μοντέλου gradient boosting (GΒ), στο πλαίσιο της πρόβλεψης του διαβήτη. Αξιοποιώντας εκτενώς τις δυνατότητες της βιβλιοθήκης Scikit-learn στην Python, αναλύουμε την απόδοση αυτών των μοντέλων χρησιμοποιώντας το σύνολο δεδομένων PIMA και διερευνούμε τον αντίκτυπο των τεχνικών κλιμάκωσης όπως η κανονικοποίηση (NormalizationNormalization), η προτοτυποποίηση(Standardization) και η μηχανική χαρακτηριστικών (Feature Engineer- τεχνικές Binning και One hot encoding). Τα ευρήματά μας αναδεικνύουν ότι το μοντέλο τυχαίου δάσους (RFRF) ως τον πιο αποτελεσματικό αλγόριθμο, επιτυγχάνοντας ακρίβεια 85% όταν συνδυάζεται με προτοτυποποίηση και μηχανική χαρακτηριστικών (Feature Engineer- τεχνικές Binning και One hot encoding). Αυτή η έρευνα συμβάλλει στον αυξανόμενο όγκο γνώσεων σχετικά με τις αναλύσεις υγειονομικής περίθαλψης που βασίζονται σε ΜΜ παρέχοντας πληροφορίες για τα δυνατά σημεία και τους περιορισμούς διαφορετικών αλγορίθμων για την πρόβλεψη του διαβήτη. Επιπλέον, η μελέτη μας προσφέρει πρακτικές οδηγίες για επαγγελματίες υγείας και ερευνητές, διευκολύνοντας την έγκαιρη ανίχνευση και εξατομικευμένες παρεμβάσεις για βελτιωμένη διαχείριση του διαβήτη. Μέσω του προσδιορισμού μελλοντικών κατευθύνσεων έρευνας, συμπεριλαμβανομένης της εξατομικευμένης ιατρικής και της βελτίωσης των μεθόδων προεπεξεργασίας, αυτή η εργασία στοχεύει να τονώσει τη συνεχή καινοτομία στον τομέα των αναλύσεων υγειονομικής περίθαλψης που βασίζονται σε ΜΜ και να προωθήσει τη διεπιστημονική συνεργασία για την αντιμετώπιση των προκλήσεων της διαχείρισης χρόνιων ασθενειών.