Μια προσέγγιση μεταφοράς γνώσης σε συστήματα συστάσεων συνεργατικού φιλτραρίσματος με μη-επικαλυπτόμενες οντότητες
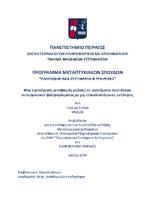
Προβολή/ Άνοιγμα
Λέξεις κλειδιά
Transfer learning ; Recommender systems ; Collaborative filteringΠερίληψη
Τα συστήματα συστάσεων χρησιμοποιούνται ευρέως στις πλατφόρμες ψυχαγωγίας και στις επιχειρήσεις ηλεκτρονικού εμπορίου για την εξατομίκευση της εμπειρίας των χρηστών και τη βελτίωση της ικανοποίησης των πελατών. Ωστόσο, αντιμετωπίζουν σημαντικές προκλήσεις, όπως το πρόβλημα της αραιότητας των δεδομένων, όπου τα δεδομένα αλληλεπίδρασης είναι αραιά, και το πρόβλημα της ψυχρής εκκίνησης (cold start), όπου νέοι χρήστες ή αντικείμενα δεν έχουν επαρκή δεδομένα αλληλεπίδρασης ώστε οι αλγόριθμοι που παράγουν συστάσεις να εκπαιδευθούν και να αποδώσουν ικανοποιητικά.
Η μεταφορά γνώσης αποτελεί μια προσέγγιση μηχανικής μάθησης, η οποία αξιοποιεί τη γνώση που αποκτάται από την επίλυση ενός προβλήματος για την ενίσχυση της λύσης ενός διαφορετικού αλλά σχετικού προβλήματος. Η εφαρμογή τεχνικών μεταφοράς γνώσης σε συστήματα συστάσεων αποσκοπεί στην αντιμετώπιση των ζητημάτων που αντιμετωπίζουν οι παραδοσιακές προσεγγίσεις επιτρέποντας την εκπαίδευση μοντέλων με λιγότερα δεδομένα και με βελτιωμένη απόδοση.
Στην παρούσα διπλωματική εργασία διερευνάται η εφαρμογή της μεταφοράς μάθησης σε συστήματα συστάσεων συνεργατικού φιλτραρίσματος με μη-επικαλυπτόμενες οντότητες. Πιο συγκεκριμένα, η μελέτη επικεντρώνεται σε σενάρια όπου δεν υπάρχουν κοινοί χρήστες ή αντικείμενα μεταξύ των τομέων, χρησιμοποιώντας μόνο τις αξιολογήσεις των χρηστών για τη μεταφορά γνώσης, χωρίς να γίνει χρήση μεταδεδομένων. Οι τομείς που χρησιμοποιούνται είναι Ταινίες και Βιβλία και τα πειραματικά αποτελέσματα αξιολογούνται με βάση την ακρίβεια της προτεινόμενης μεθόδου σε σύγκριση με παραδοσιακές τεχνικές συστάσεων που δεν κάνουν χρήση μεταφοράς γνώσης.
Αν και ο προτεινόμενος αλγόριθμος έδειξε ότι μπορεί να βελτιώσει τις συστάσεις και να μεταφέρει θετικά γνώση υπό συγκεκριμένες συνθήκες, δεν ήταν αρκετός για να ξεπεράσει σε απόδοση όλες τις παραδοσιακές μεθόδους με τις οποίες συγκρίθηκε και συγκεκριμένα την μέθοδο SVD (singular value decomposition).