Μηχανική μάθηση και ανάλυση εικόνων στον καρκίνο του εγκεφάλου
Machine learning and image analysis in brain cancer
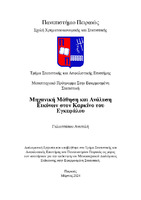
Προβολή/ Άνοιγμα
Λέξεις κλειδιά
Εγκεφαλικός όγκος ; MRI ; Transfer learning ; Convolutional neural networksΠερίληψη
Όγκος είναι μία ανώμαλη μάζα ιστού, η οποία σχηματίζεται όταν τα κύτταρα αναπτύσσονται και διαιρούνται ανεξέλεγκτα ή όταν δεν πεθαίνουν όταν πρέπει. Περισσότεροι από 150 διαφορετικοί τύποι εγκεφαλικών όγκων έχουν βρεθεί από τους ερευνητές, οι οποίοι διακρίνονται σε καλοήθεις και κακοήθεις(καρκίνος). Τόσο η ομοιότητα μεταξύ των διαφορετικών τύπων εγκεφαλικού όγκου, όσο και η ομοιότητα μεταξύ των καρκινικών και των υγιών κυττάρων που τα περιβάλλουν καθιστούν τη διάγνωση των ασθενών με εγκεφαλικό όγκο ιδιαίτερα δύσκολη. Από την άλλη μεριά τα υψηλά ποσοστά θνησιμότητας των ασθενών με κακοήθεις εγκεφαλικούς όγκους, καθιστούν αναγκαία την ανίχνευση και ταξινόμηση αυτών σε πρώιμο στάδιο. Μία τεχνική ιατρικής απεικόνισης, η μαγνητική τομογραφία MRI βοηθά τους γιατρούς να εντοπίσουν και να αναγνωρίσουν τους εγκεφαλικούς όγκους, ωστόσο λόγω όσων περιγράφηκαν παραπάνω, αυτή είναι μία ιδιαίτερα απαιτητική διαδικασία που απαιτεί χρόνο και εξειδίκευση. Η πρόοδος της μηχανικής και βαθιάς μάθησης, επιτρέπει στους ειδικούς γιατρούς την ευκολότερη και πιο αξιόπιστη διάγνωση. Οι παραδοσιακές μέθοδοι μηχανικής μάθησης, χρησιμοποιούν ορισμένες χειροκίνητες μεθόδους εξαγωγής χαρακτηριστικών για την πραγματοποίηση της ταξινόμησης των εγκεφαλικών όγκων. Αντίθετα, το πλεονέκτημα των μεθόδων βαθιάς μάθησης, όπως αυτές που χρησιμοποιήθηκαν στην παρούσα διπλωματική εργασία, είναι ότι δεν απαιτούν καμία χειροκίνητη εξαγωγή χαρακτηριστικών. Η εργασία αυτή προτείνει μία σειρά μεθόδων για την ανίχνευση και δυαδική ταξινόμηση: παρουσία και απουσία εγκεφαλικού όγκου, από ένα δημόσιο σύνολο δεδομένων, αποτελούμενο συνολικά από 3762 εικόνες MRI. Προτείνονται διάφορες προσεγγίσεις και τροποποιήσεις των μοντέλων InceptionV3, ResNet50 και MobileNetV2, με τη χρήση της τεχνικής Transfer Learning, και δύο ειδικά κατασκευασμένα συνελικτικά νευρωνικά δίκτυα(CNN) 8 και 10 επιπέδων αντίστοιχα.