Πρόβλεψη απώλειας πελατών για εμπορικές επωνυμίες καταναλωτικών αγαθών με τη χρήση δεδομένων ηλεκτρονικών συναλλαγών
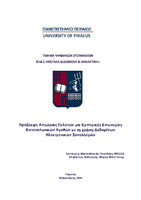
Προβολή/ Άνοιγμα
Λέξεις κλειδιά
Churn prediction ; e-Commerce ; Machine learningΠερίληψη
Η παρούσα διπλωματική εργασία ασχολείται με το πρόβλημα της Πρόβλεψης
Αποχώρησης Πελατών, εστιάζοντας στη νέα προσέγγιση της πρόβλεψης πιθανής
αποχώρησης πελατών σε επίπεδο εμπορικής επωνυμίας καταναλωτικών αγαθών στα
πλαίσια του ηλεκτρονικού εμπορίου. Ο κύριος στόχος είναι να εντοπιστούν πελάτες που είναι
πιθανό να απομακρυνθούν από μια συγκεκριμένη εμπορική επωνυμία, προσφέροντας
πολύτιμες πληροφορίες για τους κατασκευαστές προϊόντων αναφορικά με τη διαμόρφωση
μελλοντικών στρατηγικών και προωθητικών ενεργειών σε συνεργασία με τους εμπόρους
λιανικής.
Βασιζόμενη σε πραγματικά δεδομένα συναλλαγών από ένα μεγάλο διαδικτυακό
φαρμακείο του εξωτερικού, η μελέτη δεν περιλαμβάνει δημογραφικά, γεωγραφικά ή
προσωπικά στοιχεία πελατών και χρησιμοποιεί αποκλειστικά δεδομένα συναλλαγών.
Αξιοποιεί τη γλώσσα SQL για την απόκτηση δεδομένων και τη γλώσσα Python για την
κατασκευή συνόλου δεδομένων, την εκπαίδευση μοντέλων μηχανικής μάθησης και την
οπτικοποίηση των αποτελεσμάτων.
Η πειραματική μελέτη αποκαλύπτει ότι η πρόβλεψη της αποχώρησης πελατών σε επίπεδο
εμπορικής επωνυμίας είναι δυνατή, ανοίγοντας νέους δρόμους για έρευνα, οι οποίοι απέχουν
από τις συμβατικές προσεγγίσεις που απευθύνονται κυρίως σε ολόκληρες επιχειρήσεις ή
ολόκληρα ηλεκτρονικά καταστήματα. Η δοκιμή αρκετών αλγορίθμων μηχανικής μάθησης και
τεχνικών επιλογής χαρακτηριστικών οδηγεί σε ένα τελικό μοντέλο πρόβλεψης με ακρίβεια
κοντά στο 70%.
Συμπερασματικά, η παρούσα μελέτη όχι μόνο καταδεικνύει την εφικτότητα της πρόβλεψης
αποχώρησης πελατών σε επίπεδο εμπορικής επωνυμίας, αλλά προτείνει και πρακτικές
εφαρμογές, όπως η ενσωμάτωση στην πλατφόρμα eRAM. Επιπλέον, περιγράφει μελλοντικές
κατευθύνσεις για τη βελτίωση της ακρίβειας πρόβλεψης και τη διεύρυνση του εύρους της
χρησιμότητας του μοντέλου.