Πειραματική αξιολόγηση αλγορίθμων επιβλεπόμενης μηχανικής μάθησης σε δεδομένα υγείας
Evaluation of supervised machine learning algorithms on health data
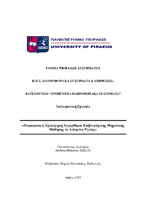
Προβολή/ Άνοιγμα
Λέξεις κλειδιά
Ηλεκτροεγκεφαλογράφημα ; Αλγόριθμοι ; Μηχανική μάθηση ; Προεπεξεργασία ; Ρύθμιση υπερπαραμέτρων ; Διασταυρούμενη επικύρωση ; Ανάλυση κυρίων συνιστωσώνΠερίληψη
Τα δεδομένα ηλεκτροεγκεφαλογραφήματος (ΗΕΓ) αποτελούν ένα μη επεμβατικό μέσο
παρακολούθησης της ηλεκτρικής δραστηριότητας του εγκεφάλου. Εδώ και χρόνια, οι επιστήμονες
και οι επαγγελματίες του ιατρικού κλάδου τα χρησιμοποιούν για να αποκτήσουν γνώσεις σχετικά με
διάφορες νευρολογικές καταστάσεις και φαινόμενα. Μια τέτοια εφαρμογή είναι και η αξιολόγηση
των οφθαλμικών καταστάσεων των ασθενών, όπου οι μικροσκοπικές αλλαγές στα μοτίβα του ΗΕΓ
μπορούν να παράσχουν πολύτιμες διαγνωστικές ενδείξεις. Τα τελευταία χρόνια η ραγδαία ανάπτυξη
των τεχνολογιών απόκτησης δεδομένων έχει οδηγήσει σε έκρηξη του όγκου των δεδομένων και,
κατ’ επέκταση, των δεδομένων ΗΕΓ που είναι διαθέσιμα για ανάλυση. Οι αλγόριθμοι μηχανικής
μάθησης (ΜΜ), μέσω των προσαρμοστικών και προβλεπτικών τους δυνατοτήτων, είναι σε θέση να
διακρίνουν μοτίβα και σχέσεις σε δεδομένα που μπορεί να διαφεύγουν από τις παραδοσιακές
αναλυτικές μεθόδους. Όταν εφαρμόζονται σωστά, όχι μόνο μπορούν να ενισχύσουν την ακρίβεια
των διαγνώσεων, αλλά και να προβλέψουν πιθανές μελλοντικές εξελίξεις. Ο συνδυασμός δεδομένων
ΗΕΓ και ΜΜ αντιπροσωπεύει ένα αναπτυσσόμενο πεδίο με ποικίλες μελέτες που εξετάζουν
αλγορίθμους από στατιστικά μοντέλα έως προηγμένα νευρωνικά δίκτυα. Η ανάδειξη της
χρησιμότητας αυτής της έρευνας στον συνδυασμό των τεχνικών ΜΜ με τα δεδομένα ΗΕΓ είναι στο
επίκεντρο της παρούσας διπλωματικής εργασίας. Συγκεκριμένα, στην παρούσα εργασία,
εφαρμόστηκε πειραματική αξιολόγηση αλγορίθμων επιβλεπόμενης ΜΜ, σε ένα σύνολο δεδομένων
ΗΕΓ που επικεντρώνεται στις καταστάσεις των οφθαλμών. Για το σκοπό αυτό, αρχικά, μελετήθηκαν
και παρουσιάστηκαν συνοπτικά τεχνικές από παρόμοιες προηγμένες έρευνες, όπου ανάλογα με τον
τομέα εφαρμογής τους διακρίθηκαν σε κατηγορίες. Στη συνέχεια πραγματοποιήθηκε εύρεση των
βέλτιστων παραμέτρων και σύγκριση των αλγορίθμων στο αρχικό σύνολο δεδομένων χωρίς
περαιτέρω προεπεξεργασία προκειμένου να αποκτηθεί ένα μέτρο σύγκρισης. Η ίδια διαδικασία
υλοποιήθηκε εκ νέου, αφού πρώτα εφαρμόστηκαν τεχνικές προεπεξεργασίας, καθώς και έγινε
παράθεση των αποτελεσμάτων και των συμπερασμάτων που προέκυψαν. Τέλος, εφαρμόστηκε η
μέθοδος ΑΚΣ για τη μείωση της διαστασιμότητας του συνόλου δεδομένων και τη διερεύνηση της
απόδοσης της ταξινόμησης μέσα από τις νέες διαστάσεις.