Evaluation of machine learning models for predicting the System Marginal Price of an Electricity System - Spanish SMP Day - Ahead forecasting
Αξιολόγηση μοντέλων μηχανικής μάθησης για την πρόβλεψη της οριακής τιμής συστήματος ηλεκτρικής ενέργειας - Περίπτωση Ισπανίας
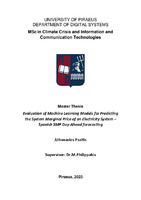
Master Thesis
Συγγραφέας
Ψάλτης, Αθανάσιος
Psaltis, Athanasios
Ημερομηνία
2023Επιβλέπων
Φιλιππάκης, ΜιχαήλFilippakis, Michael
Προβολή/ Άνοιγμα
Λέξεις κλειδιά
Machine learning ; SMP ; Spanish energy system ; Sarima ; FBProphet ; LightGBM ; Time series forecastingΠερίληψη
Η πρόβλεψη των τιμών ενέργειας στην αγορά ηλεκτρικής ενέργειας διαδραματίζει κρίσιμο ρόλο στη διασφάλιση της αποδοτικής λειτουργίας των συστημάτων ηλεκτρικής ενέργειας, βοηθώντας τους συμμετέχοντες στην αγορά να κάνουν τεκμηριωμένες επιλογές προωθώντας παράλληλα βιώσιμες πρακτικές κατανάλωσης ενέργειας. Η παρούσα διατριβή προσφέρει μια εις βάθος εξέταση τριών διαφορετικών μοντέλων πρόβλεψης χρονοσειρών: ARIMA (AutoRegressive Integrated Moving Average), Facebook Prophet και Light Gradient Boosting Machine (LightGBM). Τα μοντέλα αυτά εφαρμόζονται για την πρόβλεψη των βραχυπρόθεσμων τιμών ενέργειας (SMP) στο ισπανικό ενεργειακό σύστημα. Η έρευνα ξεκινά με την παροχή μιας επισκόπησης της αγοράς ηλεκτρικής ενέργειας στην Ισπανία, τονίζοντας τη σημασία των ακριβών προβλέψεων των τιμών για τους ενδιαφερόμενους της αγοράς, τους διαχειριστές του δικτύου και τους φορείς χάραξης πολιτικής. Επιπλέον, η διατριβή υπογραμμίζει τη σημασία της ανάλυσης, της επεξεργασίας και της προεπεξεργασίας δεδομένων για την προετοιμασία του συνόλου δεδομένων οριακής τιμής συστήματος για μοντελοποίηση. Αυτό περιλαμβάνει τεχνικές όπως η αποσύνθεση χρονοσειρών, ο έλεγχος στασιμότητας και ο μετασχηματισμός Fourier για την αποκάλυψη υποκείμενων προτύπων και τη βελτίωση της ποιότητας των δεδομένων εισόδου. Η διατριβή παρέχει επίσης, μια διεξοδική επισκόπηση κάθε μοντέλου πρόβλεψης, εξηγώντας τις θεμελιώδεις αρχές, τα πλεονεκτήματα και τους περιορισμούς τους. Στη συνέχεια εμβαθύνει στη φάση της προεπεξεργασίας των δεδομένων, παρουσιάζοντας πώς μπορούν να χρησιμοποιηθούν τεχνικές αποσύνθεσης χρονοσειρών, όπως η εποχιακή αποσύνθεση χρονοσειρών (STL), για να διαχωριστούν η τάση, η εποχικότητα και τα υπολειπόμενα στοιχεία. Χρησιμοποιούνται κριτήρια στασιμότητας όπως το κριτήριο Augmented Dickey Fuller (ADF) για να διασφαλιστεί ότι τα δεδομένα είναι κατάλληλα για μοντελοποίηση, εξασφαλίζοντας σταθερές στατιστικές ιδιότητες με την πάροδο του χρόνου. Πραγματοποιείται συγκριτική ανάλυση με τη χρήση ιστορικών δεδομένων SMP για την αξιολόγηση των επιδόσεων των μοντέλων ARIMA, Facebook Prophet και LightGBM όσον αφορά την ακρίβεια πρόβλεψης, την υπολογιστική αποτελεσματικότητα και την ευρωστία. Βασικές μετρικές όπως το μέσο απόλυτο σφάλμα (MAE) και το μέσο τετραγωνικό σφάλμα (RMSE) χρησιμοποιούνται για την αξιολόγηση των προβλεπτικών ικανοτήτων των μοντέλων. Η συγκριτική ανάλυση των μοντέλων ARIMA, Facebook Prophet και LightGBM επεκτείνεται στην αξιολόγηση της ικανότητάς τους να συλλαμβάνουν τα συστατικά, την εποχικότητα και τα μοτίβα μεταβλητότητας εντός των δεδομένων. Επιπλέον, διερευνάται η ενσωμάτωση του μετασχηματισμού Fourier για τον εντοπισμό και τη μοντελοποίηση κυκλικοτήτων στα δεδομένα, ενισχύοντας την ικανότητα των μοντέλων να καταγράφουν τις υποκείμενες περιοδικές συμπεριφορές. Αυτό αποδεικνύει την προσαρμοστικότητα των μοντέλων σε διάφορες τεχνικές χειρισμού δεδομένων. Συμπερασματικά, η παρούσα διατριβή προσφέρει μια ολοκληρωμένη προσέγγιση για την πρόβλεψη των τιμών της αγοράς ηλεκτρικής ενέργειας, ενσωματώνοντας τεχνικές ανάλυσης και χειρισμού δεδομένων με τα μοντέλα ARIMA, Facebook Prophet και LightGBM για την πρόβλεψη των τιμών SMP στην Ισπανία. Τα αποτελέσματα δίνουν τη δυνατότητα στους συμμετέχοντες στην αγορά και στους ερευνητές να επιλέγουν κατάλληλες στρατηγικές πρόβλεψης με βάση τα χαρακτηριστικά των δεδομένων, διευκολύνοντας πιο τεκμηριωμένες αποφάσεις στο δυναμικό περιβάλλον της αγοράς ηλεκτρικής ενέργειας, λαμβάνοντας παράλληλα υπόψη την εποχικότητα, τη στασιμότητα και την κυκλικότητα των δεδομένων.