Ολοκληρωμένο περιβάλλον προσομοίωσης πειραμάτων ομοσπονδιακής μηχανικής μάθησης
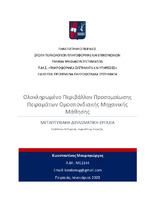
View/ Open
Keywords
Ομοσπονδιακή μάθηση ; Μηχανική μάθηση ; Ανάλυση δεδομένων ; ΑλγόριθμοιAbstract
The rapid development of the Internet of Things (IoT) has led to an exponential increase in data production. The volume of generated data is gigantic; at the same time, the rate at which they are being generated is increasing dramatically, thus leading to the Big Data era. Nowadays, there is a plethora of devices that generate their own data, which can potentially be utilized for useful information and knowledge extraction. However, the volume of data, combined with other limitations that must be taken into consideration, such as the assurance of data privacy, have led to the exploitation of alternative methods for data analysis and, in general, for the application of Machine Learning techniques. The most recent approach that has been proposed to address the aforementioned challenges is Federated Learning. Federated Learning is based on the idea of distributed data processing and analysis and the creation of Machine Learning models locally on the data source. The aforementioned individual models are then combined into a single, unified model, without transferring the data from which the respective models were derived. As a result, Federated Learning ensures the safety and reliability of data, without negatively affecting the efficiency and effectiveness of Machine Learning algorithms, compared to corresponding conventional methods. However, the choice of Federated Learning over a conventional method should be made based on different factors, such as the specific use case scenario and corresponding data, as well as the available computing resources. Taking everything mentioned above into consideration, this thesis initially provides an analytical literature review regarding Federated Learning. It then proposes an integrated framework, which provides users with the ability to run experiments with the algorithms, parameters and datasets of their liking and, finally, visualizes the results of the respective experiments in an understandable way. The objective of this specific framework is not only to act as a guide for the adoption of the Federated Learning paradigm, but also to assist in the selection of the most efficient algorithms and their parameters for a specific use case scenario.