Αυτοματοποιημένη μηχανική μάθηση για προβλήματα συσταδοποίησης
Automated machine learning for clustering problems
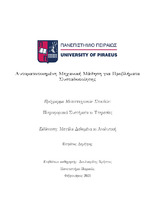
View/ Open
Abstract
The increasing availability of data and computational power, leads to the recognition of machine learning's value in many scientific and application fields. Utilization knowledge of data science's and machine learning's tools, often makes it difficult to non-experts when they want to use such tools. Automated machine learning aims to overcome this problem, by creating automation tools that can be used in a machine learning pipeline, allowing everyone to use them, without having the necessary domain knowledge.
In this thesis we deal with automated machine learning for clustering problems. Clustering problems are unsupervised machine learning problems, which means that there is no inherently external information to evaluate models' performance. For that purpose we used deep learning and meta-learning techniques to solve the problem of algorithm selection for a dataset and we approched hyperparameter optimization via bayesian optimization's tools.