Αλγόριθμοι μηχανικής μάθησης σε ανομοιογενή δεδομένα: πρόβλεψη της HIV λοίμωξης σε χρήστες ενδοφλέβιων ναρκωτικών της Αθήνας
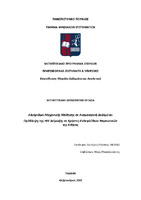
Προβολή/ Άνοιγμα
Λέξεις κλειδιά
Μη ισορροπημένα δεδομένα ; Αλγόριθμοι μηχανικής μάθησης ; HIV ; Χρήστες ενδοφλέβιων ναρκωτικώνΠερίληψη
Η μηχανική μάθηση διανύει μια περίοδο συνεχούς ανάπτυξης. Τα τελευταία χρόνια, όλο και περισσότερο, οι τεχνικές μηχανικής μάθησης χρησιμοποιούνται στην Ιατρική για διάφορα νοσήματα μεταξύ άλλων και των λοιμωδών νοσημάτων, όπως η HIV λοίμωξη. Στις αρχές του 2011 σημειώθηκε επιδημική έκρηξη της HIV λοίμωξης στον πληθυσμό των χρηστών ενδοφλέβιων ναρκωτικών (ΧΕΝ) της Αθήνας. Το Πανεπιστήμιο Αθηνών σε συνεργασία με τον Οργανισμό Κατά των Ναρκωτικών υλοποίησαν το πρόγραμμα ΑΡΙΣΤΟΤΕΛΗΣ, με σκοπό τόσο τον έλεγχο όσο και τη διασύνδεση σε φροντίδα των ΧΕΝ με HIV λοίμωξη. Σκοπός της παρούσας διπλωματικής εργασίας είναι η εύρεση βέλτιστου ταξινομητή για την HIV λοίμωξη σε ΧΕΝ της Αθήνας.
Τα δεδομένα που χρησιμοποιήθηκαν προήλθαν από το πρόγραμμα ΑΡΙΣΤΟΤΕΛΗΣ και αφορούσαν στους 3.320 μοναδικούς ΧΕΝ. Επιπροσθέτως, περιείχαν πληροφορίες για τα δημογραφικά χαρακτηριστικά, τη χρήση ουσιών, τις σεξουαλικές συμπεριφορές και τα προγράμματα μείωσης της βλάβης (προγράμματα υποκατάστασης με οπιοειδή, λήψη δωρεάν συρίγγων και άλλα). Εφαρμόστηκαν πέντε αλγόριθμοι ταξινόμησης (Logistic Regression, Random Forest, Support Vector Machines, k-Εγγύτεροι Γείτονες και Decision Tree) χρησιμοποιώντας τα δεδομένα: 1) χωρίς επαναδειγματοληψία, 2) με υποδειγματοληψία, 3) με τυχαία υπερδειγματοληψία, 4) με τεχνική υπερδειγματοληψίας συνθετικής μειονότητας και 5) με προσαρμοστική συνθετική μέθοδο δειγματοληψίας. Οι προαναφερθείσες περιπτώσεις εφαρμόστηκαν στο σύνολο των χαρακτηριστικών, ύστερα από την επιλογή μέρους αυτών καθώς και έπειτα από ανάλυση σε κύριες συνιστώσες.
Την καλύτερη επίδοση την είχε ο αλγόριθμος Random forest όταν εφαρμόστηκε σε τυχαία υπερδειγματοληψία. Η ευαισθησία, η ορθότητα καθώς και το AUC score ήταν 0.9929, 0.9805 και 0.9967, αντίστοιχα. Επιλέγοντας 34 από τα 112 χαρακτηριστικά η ευαισθησία, η ορθότητα καθώς και το AUC score ήταν 0.9929, 0.9751 και 0.9967, αντίστοιχα.
Συμπερασματικά, το αποτέλεσμα που προέκυψε από τον έλεγχο για την HIV λοίμωξη σε ΧΕΝ της Αθήνας προβλέφθηκε ορθά σε υψηλά ποσοστά, καθιστώντας τους αλγορίθμους ως ένα επιπλέον εργαλείο για την έγκαιρη ανίχνευση των οροθετικών ΧΕΝ, προκειμένου να αποφευχθεί μια νέα επιδημική έκρηξη.