Transfer learning In recommender systems
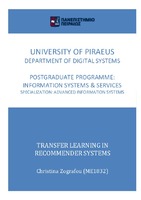
View/ Open
Keywords
Transfer learning ; Recommendation systemsAbstract
Recommendation systems are becoming more and more important nowadays providing companies with a great advantage. Data sparseness, though, is a major problem for collaborative filtering (CF) techniques in recommender systems, especially for new users and items, making it difficult to make good recommendations. That is where Transfer Learning comes in. In this study, the goal is to improve the quality of recommendations in a sparse dataset by exploiting knowledge from another dataset. More specifically, the goal is to improve the recommendations in the CDs & Vinyl domain by utilizing rating data from the Digital Music domain. Experimental results show that CDs & Vinyl recommendations can indeed benefit from making use of Digital Music recommendations.