Analytics on clinical and gene expression data to provide risk stratification predictions for cancer subtypes
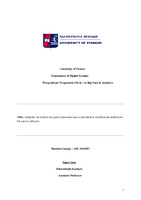
Master Thesis
Συγγραφέας
Μαρίνος, Γεώργιος
Marinos, George
Ημερομηνία
2020-02-28Επιβλέπων
Κυριαζής, ΔημοσθένηςΠροβολή/ Άνοιγμα
Λέξεις κλειδιά
Clinical data ; Genes expression data ; Cancer subtypes discovery ; Cancer subtypes identification ; Survival analysis ; Kaplan Meier curves ; Survival clusteringΠερίληψη
Η ανάλυση της επιβίωσης δεν έχει εφαρμογές μόνο για βιοϊατρικά προβλήματα για την εκτίμηση του χρόνου μέχρι την στιγμή του θανάτου και πρόσφατες μελέτες έχουν δείξει ότι είναι ένα ισχυρό εργαλείο για τη διαστρωμάτωση του κινδύνου γενικότερα. Η ανάλυση επιβίωσης είναι μια καθιερωμένη στατιστική τεχνική και τα τελευταία χρόνια υπήρξαν πολλές μελέτες που συνδύασαν την ανάλυση επιβίωσης με τεχνικές μηχανικής μάθησης και αλγόριθμους προκειμένου να επιτευχθούν καλύτερες επιδόσεις σε προβλέψεις. Επιπλέον, η χρήση διαφορετικών τύπων δεδομένων για το ίδιο υποκείμενο, αποδεικνύεται ότι βελτιώνει την απόδοση των μοντέλων μηχανικής μάθησης. Σε αυτήν τη μελέτη συνδυάζουμε κλινικά δεδομένα και δεδομένα έκφρασης γονιδίων για διαστρωμάτωση κινδύνου μιας ομάδας ασθενών με καρκίνο σύμφωνα με τους λανθάνοντες υποτύπους καρκίνου. Πρώτον, χρησιμοποιούμε κλινικά δεδομένα για την εξαγωγή χαρακτηριστικών που σχετίζονται με τον χρόνο επιβίωσης και με την παρουσία ή απουσία του συμβάντος θανάτου. Στη συνέχεια χρησιμοποιήστε αυτές τις δυνατότητες που επιλέχθηκαν για να προσδιορίσουμε τις λανθάνουσες υπο-ομάδες μεταξύ των ασθενών και έπειτα χρησιμοποιήσαμε τις ετικέτες για να προσδιορίσουμε ένα υποσύνολο σχετικών χαρακτηριστικών. Αυτή η επιλογή χαρακτηριστικών έκφρασης γονιδίων έγινε χρησιμοποιώντας τεχνικές επιλογής χαρακτηριστικών και μείωσης διαστάσεων. Τελικά εφαρμόσαμε ορισμένους ταξινομητές στο τελικό σύνολο δεδομένων και προσπαθούμε να προβλέψουμε σε ποια ομάδα ανήκει ένας μελλοντικός ασθενής. Εφαρμόζοντας αυτήν την προσέγγιση αναμένουμε ότι οι προσδιορισμένες υπο-ομάδες δεν είναι μόνο βιολογικά σημαντικές, αλλά και διαφέρουν ως προς την επιβίωση.