Μέθοδοι μελέτης του ρυθμού απώλειας πελατών και της αξίας συνολικού χρόνου ζωής πελάτη
Techniques for studying churn and customer lifetime value
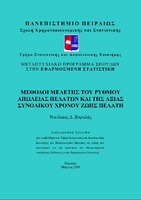
Προβολή/ Άνοιγμα
Λέξεις κλειδιά
Προβλέψεις ; Ρυθμός απώλειας ; Συνολική αξία χρόνου ζωής πελάτη ; Λογιστική παλινδρόμηση ; Γραμμικά μοντέλαΠερίληψη
Η παρούσα διπλωματική έχει ως θέμα τη πρόβλεψη του ρυθμού απώλειας και την συνολική αξία πελάτη. Τα τελευταία χρόνια πολλές επιχειρήσεις έχουν στη διάθεση τους τεράστιο όγκο δεδομένων. Τα δεδομένα αυτά μπορούν να χρησιμοποιηθούν για την δημιουργία προβλέψεων για τον ρυθμό απώλειας και συνολική αξίας πελάτη. Ωστόσο οι κλασσικές μέθοδοι που χρησιμοποιούνται μέχρι σήμερα αδυνατούν να εκμεταλλευτούν τον μεγάλο όγκο δεδομένων που είναι διαθέσιμος σήμερα.
Στόχος της εργασίας είναι αρχικά η θεωρητική περιγραφή των σύγχρονων μοντέλων μηχανικής μάθησης και έπειτα η εφαρμογή των μοντέλων σε πραγματικά δεδομένα. Συγκεκριμένα στο πρώτο κεφάλαιο γίνεται μία αναφορά στην σημασία της πρόβλεψης του ρυθμού απώλειας, συνολικής αξίας πελάτη και κλασσικές τεχνικές που χρησιμοποιούνται σήμερα. Στα επόμενα τρία γίνεται περιγραφή μοντέλων κατηγοριοποίησης, παλινδρόμησης και τεχνικές μείωσης διαστάσεων. Στο πέμπτο κεφάλαιο κατασκευάζονται μεταβλητές με σκοπό την πρόβλεψη ρυθμού απώλειας, συνολικής αξίας πελάτη, γίνεται επιλογή των σημαντικότερων και εφαρμόζονται όλες οι τεχνικές που περιγράφηκαν σε προηγούμενες ενότητες. Η σύγκριση των τεχνικών για τον ρυθμό απώλειας έγινε χρησιμοποιώντας το κριτήριο AUC και για την συνολική αξία πελάτη το κριτήριο RMSE. Το υπόδειγμα της Λογιστικής Παλινδρόμησης απέφερε τα καλύτερα αποτελέσματα για την πρόβλεψη του ρυθμού απώλειας πελάτη. Το υπόδειγμα LightGBM είχε καλύτερη προσαρμογή από ότι η τεχνική Random Forest το οποίο επιβεβαιώνει την χρησιμότητας της.