Σταδιακή συλλογή και ανάλυση δεδομένων με εφαρμογή στη διαχείριση και τον έλεγχο κυβερνοφυσικών συστημάτων υγείας
Incremental data collection and analysis applied to the management and control of health cyber physical systems
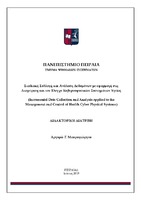
View/ Open
Keywords
Διαδίκτυο των Αντικειμένων ; Ετερογένεια ; Internet of Things ; Αξιοπιστία ; Κυβερνοφυσικά συστήματα ; Ενσωμάτωση συσκευών ; Cyber Physical Systems (CPS) ; Συλλογή δεδομένωνAbstract
The internet represents a digital space where big amounts of information, services, and data are added and exchanged daily, influencing and transforming the way in which people interact and communicate with each other. The great capabilities of the internet in conjunction with the relevant developments in the speed of the data recording and retrieval, have enabled the creation of new intelligent systems that offer increased efficiency, productivity, security and speed. To this context, the Cyber-Physical Systems (CPS) have developed, where the digital and the physical systems are now able to communicate directly with each other by recording the information contained in physical devices, ensuring their safe, efficient and intelligent operation. In order to achieve that, CPS utilize their different layers of sensors/actuators, communication, and applications so as to be able to cope with the emerging needs and requirements. This layer of communication is also the point where the combination of the CPS technologies and the Internet of Things (IoT) technologies emerges. IoT is a very powerful part of CPS, which is growing at a rapid pace and includes a variety of devices (e.g. smartphones, tablets, portable devices, sensors, cameras, etc.). It is reported that out of the 19.4 billion connected devices in 2019, 8 billion of them are referred to IoT connected devices, a number that is expected to rapidly increase over the next few years, producing large volumes of data. This fact strengthens the vision for developing new communication technologies and finding new ways in order to be able to synchronize all these huge amounts of the existing devices. However, this vision is accompanied by a number of different challenges, such as the fact that all these devices produce data that often do not fit to the nature of the platforms that collect them, thus being unable to be successfully analyzed by these platforms, in order to produce the acquired useful knowledge and insights. Consequently, there is a great need to develop new solutions for the adaptive selection, management and analysis of all of this produced and existing data that is produced by the heterogeneous device.
However, in order to address this challenge, it is assumed that all this data has been successfully collected by the existing underlying heterogeneous devices. Therefore, addressing the challenge of integrating devices and collecting their data needs to be resolved before tackling the difficult task of successfully analyzing and exploiting the data. IoT, however, is limited to monitoring and controlling all existing enormous quantities of existing devices, as emerging support technologies that need to support these devices, have to predict not only this dramatic increase in connected devices but also their heterogeneity since they have different specifications, capabilities and functions. Thus, it is necessary to adopt new technologies for automatically recognizing and understanding the nature of all the existing IoT devices in order to become feasible to automatically collect and analyze the data that is generated by these devices. However, existing management and analysis techniques are able neither to cope with the complexity of the devices nor to recognize the nature of these devices, as they are both static and sensitive to new or changing changes of the functions and the requirements of the existing devices. Henceforth, what is needed is a generalized automated approach that will be able to connect and integrate heterogeneous devices, provided as a basis for efficiently retrieving data from all the underlying heterogeneous devices. In addition, since IoT devices are typically characterized by a high degree of heterogeneity, they are recognized as reliable at different levels, thus providing data of different levels of reliability. Therefore, the research challenge that emerges is on the one hand the difficulty of managing all these huge amounts of heterogeneous devices that include their own specifications and interfaces, and on the other hand the need for the devices themselves and the data that is produced by them to be fully reliable. Towards this direction, it is needed both an automated effective way for connecting and integrating the heterogeneous devices into different IoT platforms so as to collect their data, and an automated effective way for measuring the reliability of these devices in combination with the reliability of their produced data.
In order to effectively address all of the aforementioned challenges, the current Ph.D. thesis focuses on them, having as a primary scope to study, design and implement a model of integrating data that is received from all the different autonomous and heterogeneous IoT devices, of both known and unknown nature. For this purpose, a general plug’n’play devices approach is proposed for the automatic management of heterogeneous IoT devices, estimating their levels of reliability, and finally collecting data only from the reliable and relevant ones to each platform. This approach is implemented through an innovative interoperable mechanism that can both connect to different IoT platforms and facilitate the automatic recognition, interaction and access to all underlying heterogeneous devices. In particular, the mechanism consists of the four (4) stages of the techniques of the device discovery and connection, the device type recognition, the device data collection, and the device and data quality estimation. Through this process, the proposed mechanism is able to achieve three (3) distinct goals. First of all, through this mechanism it becomes feasible to identify the nature of the devices that are available each time for connection to the corresponding platforms, thus giving the ability to these platforms to be connected only with the devices that are associated with them, in the sense that their data are relevant and useful to the nature of these platforms. Apart from this, the mechanism can automatically collect the data of the connected devices, thereby enabling the interoperability of each corresponding platform, without needing it to have further knowledge of the interfaces of these devices, and thus without needing to be parameterized in order to be able to collect the data of these devices. Finally, the mechanism is able to automatically evaluate the reliability of both the connected devices and their generated data, thereby enabling each corresponding platform to use and exploit only the reliable and qualified data that is produced by only trusted devices. As a result, it minimizes the risk of producing wrong results’ reports, and the potential handling error actions that could emerge under erroneous and incomplete data. The mechanism was evaluated through various experiments on different scenarios in the healthcare domain, producing quite reliable results, thus indicating its feasibility and applicability in this domain. However, this mechanism can be widely used and suited in many different domains, except of the healthcare domain, since it addresses the challenge of the optimal and efficient use of IoT devices regardless of their use in a specific application domain.