Ανίχνευση ασυνήθιστης συμπεριφοράς στην εξόρυξη δεδομένων
Anomaly detection in data mining
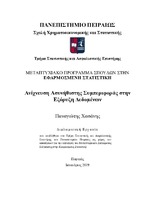
Προβολή/ Άνοιγμα
Λέξεις κλειδιά
Anomaly detection ; Outlier detectionΠερίληψη
Ο όρος ανίχνευση ασυνήθιστης συμπεριφοράς ή ανωμαλιών χρησιμοποιείται για να περιγράψει τεχνικές με τις οποίες εντοπίζονται αντικείμενα, γεγονότα ή παρατηρήσεις οι οποίες δεν ακολουθούν τους γενικούς κανόνες των δεδομένων που παρατηρούμε και χαρακτηρίζονται ως ανωμαλίες. Οι ανωμαλίες προκύπτουν εξαιτίας μηχανικών βλαβών, αλλαγές στη συμπεριφορά του συστήματος, δόλια συμπεριφορά, ανθρώπινο σφάλμα, σφάλμα οργάνου ή απλά μέσω φυσικών αποκλίσεων στους πληθυσμούς. Η έγκαιρη ανίχνευση των ανωμαλιών μπορεί να εντοπίσει τα σφάλματα του συστήματος και την απάτη πριν κλιμακωθούν με δυνητικά καταστροφικές συνέπειες.
Πολλές τεχνικές ανίχνευσης ασυνήθιστης συμπεριφοράς έχουν αναπτυχθεί ειδικά για ορισμένους τομείς εφαρμογής, ενώ άλλες είναι πιο γενικές. Στη παρούσα διπλωματική εργασία προσπαθούμε να παρέχουμε μια δομημένη και ολοκληρωμένη επισκόπηση της έρευνας σχετικά με την ανίχνευση ασυνήθιστης συμπεριφοράς. Για τις διαφορετικές κατηγορίες τεχνικών ανίχνευσης ανωμαλιών παρουσιάζεται η βασική τεχνική και οι παραδοχές, οι οποίες χρησιμοποιούνται για τη διαφοροποίηση μεταξύ κανονικής και ανώμαλης συμπεριφοράς. Επίσης, για κάθε κατηγορία παρουσιάζονται διάφορες μέθοδοι ανίχνευσης ανωμαλιών, οι οποίες στηρίζονται στη αντίστοιχη βασική τεχνική, και προσδιορίζονται τα πλεονεκτήματα και τα μειονεκτήματά τους. Τέλος, γίνεται εφαρμογή και σύγκριση της απόδοσης διάφορων μεθόδων ανίχνευσης ασυνήθιστης συμπεριφοράς.