Personalized software tutoring and affective human-computer interaction in social networking-based language and open learning
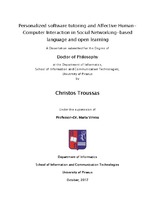
Doctoral Thesis
Συγγραφέας
Troussas, Christos
Τρούσσας, Χρήστος
Ημερομηνία
2017-10Επιβλέπων
Βίρβου, ΜαρίαΠροβολή/ Άνοιγμα
Λέξεις κλειδιά
Web-based tutoring ; Intelligent tutoring systems ; Tutoring of foreign languages ; e-Learning ; Computer-Supported Collaborative Learning (CSCL) ; Intelligent Computer-Assisted Language Learning (ICALL)Περίληψη
H παρούσα διδακτορική διατριβή παρουσιάζει μία καινοτόμο προσέγγιση διαδικτυακής και εξ αποστάσεως εκπαίδευσης, προσφέροντας εξατομίκευση στις ανάγκες των μαθητών. Το υλοποιημένο ευφυές σύστημα διδασκαλίας, που ονομάζεται POLYGLOT, ενσωματώνει χαρακτηριστικά κοινωνικών δικτύων στο εκπαιδευτικό περιβάλλον διεπαφής. Αυτά τα χαρακτηριστικά περιλαμβάνουν την ανάρτηση σε «τοίχο», αναφορά ονόματος χρήστη στον «τοίχο» με χρήση ετικέτας, σύγχρονη και ασύγχρονη επικοινωνία με χρήση κειμένου, κουμπιά αντίδρασης («Μου αρέσει», «Δε μου αρέσει») σε ερωτήσεις και δήλωση συναισθηματικής κατάστασης. Επιπλέον, το σύστημα POLYGLOT προσφέρει και ένα εργαλείο συγγραφής με το οποίο οι διδάσκοντες μπορούν να αλλάζουν το εκπαιδευτικό υλικό και να ελέγχουν την πρόοδο των μαθητών.
Δεδομένου ότι το πεδίο γνώσης του συστήματος POLYGLOT είναι η εκμάθηση γραμματικών φαινομένων στην Αγγλική και Γαλλική γλώσσα, χρησιμοποιείται η θεωρία απόκτησης δεύτερης γλώσσας του Stephen Krashen, η οποία αποτελείται από τις ακόλουθες υποθέσεις: Υπόθεση Απόκτησης-Εκμάθησης, Υπόθεση Επίβλεψης, Υπόθεση Δεδομένων Εισόδου, Υπόθεση Φυσικής Πορείας και η Υπόθεση Συναισθηματικού Φίλτρου. Έτσι, η εκμάθηση μέσω του συστήματος POLYGLOT συμβαδίζει πλήρως με την προαναφερθείσα θεωρία αναφορικά με τον τρόπο διδασκαλίας, τον τρόπο συνεργασίας, τους χρονικούς περιορισμούς στην εκμάθηση, την διατήρηση αρχείων μαθητών, την λογική διαβάθμιση των εννοιών διδασκαλίας και την αντίδραση στην απογοήτευση των μαθητών με τη μορφή ενθαρρυντικών μηνυμάτων.
Προς την κατεύθυνση της εξατομικευμένης διδασκαλίας, το σύστημα POLYGLOT αυτόματα αναγνωρίζει την προτίμηση τρόπου μάθησης των σπουδαστών. Η προτίμηση του τρόπου μάθησης των σπουδαστών βασίζεται στο μοντέλο Felder-Silverman και το σύστημα POLYGLOT κατατάσσει τους μαθητές ως ενεργητικούς ή στοχαστικούς και ακολουθιακούς ή ολιστικούς. Οι ενεργητικοί μαθητές προτιμούν να επικοινωνούν με τους συμμαθητές του και να μαθαίνουν μέσα από τη συνεργασία με κάποιο συμμαθητή ώστε να συζητούν για το υλικό διδασκαλίας. Εν αντιθέσει, οι στοχαστικοί μαθητές προτιμούν να μη συνεργάζονται και να διαβάζουν μόνοι τους. Οι ακολουθιακοί χρήστες προτιμούν να διαβάζουν μόνοι. Οι ακολουθιακοί μαθητές προτιμούν να διαβάζουν σταδιακά και προοδευτικά, έχοντας μια γραμμική πορεία εκμάθησης. Από την άλλη πλευρά, οι ολιστικοί μαθητές προτιμούν να πλοηγούνται στο υλικό διδασκαλίας από κεφάλαιο σε κεφάλαιο με τυχαίο τρόπο. Η αυτόματη αναγνώριση της προτίμησης του τρόπου μάθησής τους γίνεται με τη χρήση ενός αλγορίθμου επιτηρούμενης μάθησης, του αλγορίθμου πλησιέστερου γείτονα, ο οποίος λαμβάνει σαν είσοδο χαρακτηριστικά χρηστών, όπως η ηλικία του, το φύλο τους, το μορφωτικό τους επίπεδο, το επίπεδο γνώσης υπολογιστών, τις γλώσσες που ομιλούν και το βαθμό τους σε ένα προκαταρκτικό διαγώνισμα.
Επιπρόσθετα, το παρουσιαζόμενο μοντέλο μαθητή ενσωματώνει ένα μηχανισμό εντοπισμού και διάγνωσης λαθών, το οποίο συνδυάζει δύο αλγοριθμικές προσεγγίσεις σε ένα υβριδικό μοντέλο με σκοπό να βρει την αιτία των λαθών των μαθητών. Η πρώτη τεχνική είναι η κατά προσέγγιση αντιπαραβολή συμβολοσειρών η οποία βρίσκει προσεγγιστικά υποσυμβολοσειρές που ταιριάζουν με κάποιο μοτίβο και διαγιγνώσκει λάθη, όπως λάθη απροσεξίας, λάθη στην αντωνυμία, λάθη ορθογραφίας και λάθη στους χρόνους των ρημάτων. Η δεύτερη τεχνική είναι η σήμανση ομοιότητας συμβολοσειρών, η οποία διαγιγνώσκει λάθη που οφείλονται στη χρήση γνώσης από μία γλώσσα στην άλλη.
Επίσης, το σύστημα POLYGLOT ενσωματώνει ένα μοντέλο για τη συνεργασία των μαθητών, η οποία θα είναι ωφέλιμη και για τις δύο πλευρές. Πιο συγκεκριμένα, η πρόταση για συνεργασία αφορά δύο περιπτώσεις. Στην πρώτη περίπτωση, το σύστημα προτείνει συνεργασία μεταξύ δύο μαθητών που έχουν συμπληρωματική γνώση, δηλαδή αν ο μαθητής 1 έχει υψηλή γνώση στο αντικείμενο Α και χαμηλή γνώση στο αντικείμενο Β θα του προταθεί συνεργασία με το μαθητή 2 που έχει υψηλή γνώση στο αντικείμενο Β και χαμηλή γνώση στο αντικείμενο Α. Αντίστοιχα, στη δεύτερη περίπτωση προτείνεται συνεργασία βάσει λαθών, δηλαδή μεταξύ μαθητών εκ των οποίων ο μαθητής 1 κάνει λάθη της κατηγορίας Α αλλά όχι λάθη της κατηγορίας Β και ο μαθητής 2 κάνει λάθη της κατηγορίας αλλά όχι λάθη της κατηγορίας Α. Αυτή η λογική μπορεί να βοηθήσει τους μαθητές στην εκπαιδευτική διαδικασία και να βελτιώσει τους βαθμούς απόκτησης και αποκατάστασης γνώσης.
Στο σύστημα POLYGLOT, οι μαθητές έχουν τη δυνατότητα να δηλώσουν τη συναισθηματική τους κατάσταση μεταξύ των «Χαρούμενος», «Απογοητευμένος» και «Ουδέτερος». Παρ’ όλα αυτά, η αλληλεπίδραση με το σύστημα, δηλαδή η αντιμετώπιση δυσκολιών σε διαγώνισμα ή ένας κακός βαθμός, μπορεί να αποτελέσει εμπόδιο στην επίτευξη των στόχων του, το οποίο με τη σειρά του προκαλεί το αρνητικό συναίσθημα της απογοήτευσης. Το σύστημα POLYGLOT μπορεί να εντοπίσει την απογοήτευση του μαθητή, χρησιμοποιώντας το μοντέλο γραμμικής παλινδρόμησης. Οι σχέσεις μοντελοποιούνται χρησιμοποιώντας λειτουργίες γραμμικής πρόβλεψης των οποίων οι άγνωστες παράμετροι του μοντέλου υπολογίζονται από τα δεδομένα.
Τελικώς, η αντίδραση του συστήματος POLYGLOT στην απογοήτευση των μαθητών είναι η προβολή ενθαρρυντικών μηνυμάτων, βασισμένων στη θεωρία απόδοσης ενθαρρυντικών μηνυμάτων (θεωρία απόδοσης αιτιών), η οποία περιλαμβάνει τρία στάδια και υπογραμμίζει ότι η συμπεριφορά πρέπει να παρατηρείται, πρέπει να καθορίζεται ως σκόπιμη και να αποδίδεται σε εσωτερικές ή εξωτερικές αιτίες. Με τη χρήση των ενθαρρυντικών μηνυμάτων, οι μαθητές βοηθούνται κατά την εκπαιδευτική διαδικασία και αποθαρρύνονται από τη διακοπή της μελέτης.
Όλες οι προαναφερθείσες προσεγγίσεις έχουν υλοποιηθεί, εφαρμοστεί και αξιολογηθεί πλήρως. Το σύστημα POLYGLOT χρησιμοποιήθηκε από τους μαθητές ενός φροντιστηρίου ξένων γλωσσών στην Αθήνα, προκειμένου να διδαχθούν τα γραμματικά φαινόμενα και στις δύο γλώσσες. Για την αξιολόγηση όλων των λειτουργιών του συστήματος POLYGLOT, χρησιμοποιήθηκε το μοντέλο τεσσάρων επιπέδων του Kirkpatrick. Τα αποτελέσματα της αξιολόγησης ήταν πολύ ενθαρρυντικά. Απέδειξαν ότι το σύστημα αποτελεσματικά προσαρμόζει την εκπαιδευτική διαδικασία στην προτίμηση του τρόπου μάθησης των σπουδαστών, καθώς επίσης τους βοηθάει στη διάγνωση των λαθών τους, προτείνει συνεργασίες ωφέλιμες και για τους δύο μαθητές, εντοπίζει την απογοήτευσή τους και απαντά σε αυτή.