Αξιολόγηση αλγορίθμων συσταδοποίησης ακολουθιακών χωροχρονικών δεδομένων με χρήση διαφορετικών συναρτήσεων απόστασης
Evaluation of clustering algorithms of sequential spatio-temporal data with various distance functions
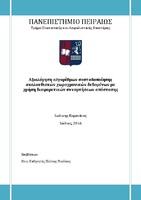
Προβολή/ Άνοιγμα
Λέξεις κλειδιά
Συναρτήσεις ομοιότητας ; Μετρικές συναρτήσεις απόστασης ; Συσταδοποίηση ; Αλγόριθμοι ; ΣυστάδεςΠερίληψη
Η παρούσα διπλωματική εργασία έχει ως βασικό στόχο τη μελέτη και την αξιολόγηση της απόδοσης αλγορίθμων συσταδοποίησης ακολουθιακών χωροχρονικών δεδομένων, χρησιμοποιώντας κάθε φορά διαφορετικά μέτρα απόστασης/ομοιότητας με σκοπό την ανάδειξη των πλεονεκτημάτων και των μειονεκτημάτων τους. Συγκεκριμένα, εφαρμόζονται μετρικές συναρτήσεις απόστασης (Ευκλείδεια, Manhattan, Chebyshev, Ευκλείδια STARTEND), και μη μετρικές συναρτήσεις απόστασης είτε βασισμένες στη δυναμική χρονική στρέβλωση (Dynamic Time Warping), είτε βασισμένες στην "επεξεργασία" της απόστασης (Edit Distance on Real sequence) ή βασισμένες στη μεγαλύτερη κοινή υποαλληλουχία (Longest Common Subsequence). Ποικίλοι μετασχηματισμοί τροχιών (επαναδειγματολειψία, προσθήκη θορύβου και μετατόπιση σημείου) ελεγχόμενοι από δυο παραμέτρους, τον ρυθμό και την απόσταση, εφαρμόζονται σε πραγματικά και συνθετικά σύνολα δεδομένων τροχιών. Για κάθε μετασχηματισμό, αξιολογείται η ομαδοποίηση του αρχικού συνόλου δεδομένων και των μετασχηματισμένων συνόλων δεδομένων ανάλογα με την τιμή της παραμέτρου που "τρέχει". Τα εξαγόμενα αποτελέσματα της εκτενούς πειραματικής μελέτης χρησιμοποιούνται για την αξιολόγηση της εγκυρότητας των ομαδοποιήσεων που επιτυγχάνονται από τον αλγόριθμο optics και την ιεραρχική ομαδοποίηση με τη μέθοδο Ward, αντίστοιχα.