Τεχνικές ανάλυσης πολυδιάστατων οικονομικών χρονοσειρών
Analysis of multivariate financial time series
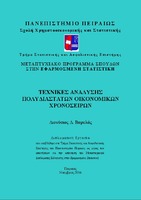
View/ Open
Keywords
Μέθοδοι πρόβλεψης ; Χρονοσειρές ; Ακαθάριστο Εθνικό Προϊόν ; ΑΕΠ ; Δυναμικό μοντέλο ανάλυσης παραγόντων ; Μοντέλο χώρου καταστάσεωνAbstract
In recent years many institutions and research centres have at their disposal large datasets.
Those data can be exploited in order to obtain more accurate forecasts of various economic
data. However, traditional methods of multivariate time series analysis cannot exploit the
large volume of data due to the large number of variables compared to the number of observations.
That problem can be overcome by the use of a dynamic factor model, which creates new
variables as linear functions of the original variables. Those new variables can summarize
effectively the information held by the original ones.
The aim of this thesis was firstly to theorytically describe one of the available dynamic
factor models and secondly to apply this methodology on a greek dataset. In particular, we
have constructed a model which predicts GDP and we have compared that model to an
ARIMA model. The comparison was made using the criteria MSFE, MAE and MAPE. The
dynamic factor model was estimated in two steps. In the first step we extracted the factors
using classic factor analysis. In the second step those factors were an important part of the
state-space model used in order to obtain forecasts. The fitting of that model was better
compared to the fitting of the ARIMA model, which confirms the fact that dynamic factor
models can be useful in forecasting economic data.