Behavioural modeling in online auctions using data analysis
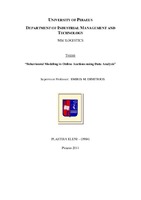
Θεματική επικεφαλίδα
Internet auctions ; Auctions -- Computer network resources ; Πλειστηριασμοί ; Ηλεκτρονικό εμπόριο -- Δίκαιο και κανονισμοίΠερίληψη
The present work deals with the investigation of the behavioural aspects of
bidders in electronic auctions. The subject is of interest as it has been noticed that
behavioural patterns exist in auctions of sibling items. This has triggered many
research attempts, which employ different approaches in modeling these behaviours.
Modeling human behaviour is of interest as the decision making process of a
bidder of whether and when to place a bid, may not be subject to rationality; bounded
rationality asserts that decision-makers are intendedly rational; that is, they are goaloriented
and adaptive, but because of human cognitive and emotional architecture,
oftentimes, rationality fails and as a consequence, there is a mismatch between the
decision-making environment and the choices of the decision-maker .
This research approaches the problem at hand using splines (cubic
polynomials) to fit curves between knot points that have been collected from
numerous auctions. As a testbed the electronic platform of eBay is used, while as
family of auctioned items, without loss of generality, the family of antique maps and
engravings has been selected; then, these are classified per auction duration, that is in
5, 7 and 10 days. The results extracted show that: (i) auctions with the same duration
exhibit uniform characteristics, and (ii) no significant differences are noticed when
duration changes. Also our approach is evaluated in other types of items and the
results are encouraging and justify our decision to model behaviour using splines.
The aim of this work is to explore whether behaviour can be modeled and
whether the polynomial approach is adequate. In the first place a second order
polynomial is used, which proves to suffer from insufficiencies in modeling
behaviour, which justifies the proceeding to use cubic polynomials (splines). Splines,
in general, are very commonly used in curve fitting, due to their excellent properties
in smooth curves (continuity in 1st and 2nd derivative); in statistics, to smooth a data
set is to create an approximating function that attempts to capture important patterns
in the data, while leaving out noise or other fine-scale structures/rapid phenomena.
Curve fitting concentrates on achieving as close a match as possible between any two
points that the order of equation involves; curve fitting implies that regression
analysis is used, so that it may be attempted to explain how the typical value of the
dependent variable changes when any one of the independent variables is varied,
while the other independent variables are held fixed.