The accounting treatment of research & development expenditures for financial firm performance : a machine learning approach
Η λογιστική αντιμετώπιση των δαπανών έρευνας και ανάπτυξης για την χρηματοοικονομική απόδοση των εταιρειών : μια προσέγγιση με μηχανική μάθηση
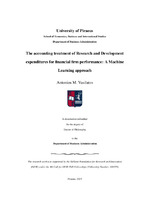
Doctoral Thesis
Author
Vasilatos, Antonios M.
Βασιλάτος, Αντώνιος Μ.
Date
2025-01-08View/ Open
Keywords
R&D costs ; Machine learning ; Financial firm performance ; IAS 38Abstract
This thesis analyses the predictive ability of R&D expenditures, on future firm financial performance, utilizing a European sample of publicly listed firms that reported under IFRS from 2005 to 2020. This study employs a forward-looking, out-of-sample predictive approach, contrasting with prior research that predominantly examines the relationship between R&D expenditure and subsequent profitability through in-sample regression analysis. This re-frames the enduring argument on R&D accounting treatment—whether to capitalize or expense—as a forecasting issue, coinciding with contemporary literature advocating for predictive analysis over solely explanatory methods.
Machine learning algorithms are employed, such as, logistic regression, random forests, SVM and XGBoost, to predict one-step-ahead profitability measures such as ROA, Price, Returns, and EPS. This work departs from conventional econometric modeling, which focuses on parameter estimation, and illustrates the ability of machine learning algorithms to identify non-linear correlations and produce more precise out-of-sample predictions. Furthermore, it presents a data-driven approach by comparing theoretically produced financial ratios with models constructed from raw accounting data. Remarkably, the latter frequently demonstrate comparable, if not superior, predictive ability for future firm financial performance.
To gain insights into the contribution of R&D expenditures to future firm performance, feature importance metrics and SHAP (SHapley Additive exPlanations) values are used to improve the interpretability of ML models, offering detailed insights into the impact of capitalized vs expensed R&D expenditures on predictive accuracy. Despite previous findings that capitalization promotes earnings manipulation and diminishes predictive accuracy, the results demonstrate that capitalized R&D costs can enhance future financial performance predictions. This indicates that the existing accounting treatment under IFRS, which allows for the capitalization of development expenditures under certain conditions, may more effectively communicate the information required by investors and policymakers to forecast future firm financial performance.
This research contributes to the accounting and finance literature by providing substantial out-of-sample evidence about the predictive significance of R&D expenditures. This study offers methodological guidance for incorporating machine learning techniques into accounting research and explains the contentious topic of R&D capitalization, potentially advising standard setters, practitioners, and investors on the optimal interpretation and application of R&D information for prospective decision-making.