Εφαρμογή των δικτύων Kolmogorov-Arnold (KAN) στην πρόβλεψη πιστωτικού κινδύνου
Application of Kolmogorov-Arnold networks (KAN) in credit risk forecasting
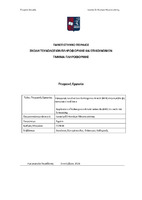
Bachelor Dissertation
Author
Ελ-Χαττάμπ-Μπιστογιάννης, Ιωακείμ
Date
2024-09Advisor
Σωτηρόπουλος, ΔιονύσιοςView/ Open
Keywords
Πρόβλεψη πιστωτικού κινδύνου ; Δίκτυα Kolmogorov-Arnold (KAN) ; Ενίσχυση κλίσης ; Λογιστική παλινδρόμηση ; Πιθανότητα αθέτησης ; Credit risk prediction ; Kolmogorov-Arnold Networks (KAN) ; Gradient boosting ; Logistic regression ; Default probabilityAbstract
Credit risk prediction is one of the most critical factors in the operation of financial institutions, as it is directly linked to their ability to assess the risk of default by clients and make strategic decisions regarding loan approvals and capital management. Accurate credit risk prediction enables effective capital allocation, minimizes losses, and enhances the stability of the financial system.
This thesis explores the application of Kolmogorov-Arnold Networks (KAN) in credit risk prediction, comparing its effectiveness with two widely used techniques in this field: gradient boosting and logistic regression. The study utilizes the Home Credit Default Risk dataset from Kaggle to analyze the performance of these three techniques in predicting default probability.
The results indicate that the KAN method outperforms logistic regression but falls short compared to gradient boosting. While KAN achieved satisfactory results, it requires further optimization to compete effectively with more advanced techniques like gradient boosting, which proved superior in credit risk prediction. The findings suggest that KAN has potential as a promising method in this field, with significant room for improvement.