Εφαρμογή και ανάλυση στην αναγνώριση εικόνων με τη χρήση «Coral Tensor Processing Unit»
Performance analysis of the accelerator "Coral Tenser Processing Unit" in picture recognition
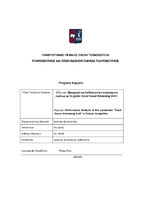
View/ Open
Keywords
Coral TPU ; Neural network ; Artificial intelligence ; Machine learning ; Object recognition ; Artificial neural networks ; Complex neural structures ; Training algorithms ; Machine learning accelerators ; Tensor Flow Lite ; TPU technologyAbstract
Artificial Intelligence is one of the fastest-growing fields in Computer Science. The problem that this work solves belongs to the area of modern neural networks. Modern neural networks manage complex processes and therefore require a lot of computing power. Modern general-purpose processors "struggle" to achieve adequate and satisfactory performance. Google developed Tensor Processing Units (TPUs) to accelerate the execution of AI applications in data centers and other applications, fulfilling this purpose. In this thesis, we deal with Edge TPU. The Edge TPU is a small integrated circuit that enables the development of IT applications "at the edge". The Edge TPU can perform four (4) trillion operations per second, using 2 Watts of power. However, the architecture and instruction set of such AI accelerators present various challenges and limitations. We benchmarked the TPU, with ready-made models provided by Google, to evaluate its capabilities. The obtained results reveal a significant speedup for Google Edge TPU compared to BCM 2837 (Raspberry Pi 3, A+), and AMD Ryzen 5 3500U processors. Overall, a significant speedup of large-scale convolutional neural networks and simple artificial neural networks is achieved. Edge TPU provides up to 10x better performance than BCM 2837 and 7x better performance than AMD Ryzen 5 3500U.