Μέτρα εξάρτησης και υποφαινομενικές συμπεριφορές για γραμμικές σχέσεις που δημιουργούνται από μη-γραμμικής μορφής χρονοσειρές
Measures of dependence and spurious behaviors for linear relationships generated from non-linearly structured time series
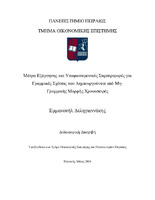
View/ Open
Keywords
χρονοσειρές ; συντελεστής συσχέτισης ; μέτρα εξάρτησης ; υποφαινομενικές σχέσεις ; Copulas ; AR(1) – ARCH(1) ; Time series ; Correlation coefficient ; Measures of dependence ; Spurious relationshipsAbstract
Determining the relationship between two variables is one of the most crucial aspects of any quantitative analysis. The goal of this effort is to explore if there is, and to what extent, a dependency between the two variables so that the analysis can expand to quantitatively determine their relationship. The technique of correlation coefficient commonly used in quantitative analysis has often been proven to be insufficient in adequately identifying the existence of variable relationships, given that the value of the correlation coefficient depends on the nature of the data, showing sometimes spurious relationships in the case of independent time series. For this reason, more complex techniques, such as Copulas, are used to measure dependency in a better way, capturing the concept of dependency between variables even for data with high variability and diversity.
This doctoral thesis investigates the behavior of various dependency measures, including the correlation coefficient, for linear relationships generated by non-linear time series with significant variability. Specifically, the AR(1) - ARCH(1) models introduced by Bera, Higgins, and Lee (1992 and 1996) are used for the simulation application, which exhibit high variability in their values, as heteroskedasticity stems from the variable values rather than from the random error values. Moreover, as the authors state, these models can also be presented as non-linear models with time-varying coefficients.
The first chapter briefly outlines the basic elements used in the analysis of random variables and describes their behavior. The concept of the joint distribution of two random variables is then analyzed with the aim of presenting the linear correlation coefficient, while there is even a brief mention of the problem of spurious correlations that arise from the use of stationary and non-stationary time series.
In the second chapter, the basic concepts of Copula functions are presented along with Sklar's theorem, which establishes the existence of these functions and defines their upper and lower bounds. Subsequently, the most important and widely used Copula functions are described which will be applied in this doctoral thesis to explore their behavior in complex time series, with a brief reference at the end on how to estimate their parameters.
In the third chapter, the characteristics and properties of stationary time series are introduced along with the basic concepts of the Box and Jenkins methodology. Then, non-stationary time series are described along with their corresponding statistical tests used to detect the presence of unit autoregressive root, noting that these time series reflect behaviors of phenomena with significant variability. Within this context, conditional heteroskedasticity models are presented, which depict variability in a different way, where the fluctuation of time series values changes over time with a specific pattern. Finally, the Bera, Higgins, and Lee (1992 and 1996) models are presented as another form of models that may include even greater variability in time series values.
In the fourth chapter, the behavior of the correlation coefficient and various Copula techniques for independent, linearly dependent time series with heteroskedasticity is explored, where the behavior of some of them resembles non-linear behavior, using Monte Carlo analysis. Additionally, the correlation behavior that arises from such models is investigated based on the statistical tests for the null hypothesis that there is no linear correlation along with the power of the test to understand better the spurious correlation phenomenon as well as the behaviors of the dependency measures used in this research.
In the fifth chapter, a Monte Carlo analysis is applied to explore the phenomenon of spurious regression for two independent stationary first-order autoregressive AR(1) time series, as well as two independent non-stationary random walk time series, with errors that do not have constant variance. Indeed, the errors were constructed by a time-varying variance following an autoregressive conditional heteroskedasticity first-order model, namely an ARCH(1) model, where the variability of the variance arises either from error values or from the time series values themselves, with the latter forming a family of time series that can also be considered as non-linear processes. The error structures of this regression analysis are also investigated to detect serially correlated errors along with an effort to show how to address both issues.
In the sixth and final chapter, the dependency relationships between various variables related to the energy market are examined using all the Copula techniques applied in this thesis. Specifically, the effort is consecrated on the price of oil for several other macro and finance variables for a specific period. Initially, the relationships of all variables are examined in their levels and then in their first differences, given that these time series were found to be first-order non-stationary processes. Finally, the analysis of their dependency is extended to the fitted values as well as the residuals resulting from the estimation of the most suitable ARIMA model, noting significant changes in their relationships.