Συσχέτιση ναυτιλιακών δεδομένων και δεδομένων προωστήριας εγκατάστασης με χρήση προγραμμάτων τεχνητής νοημοσύνης για την πρόβλεψη της κατανάλωσης καυσίμου
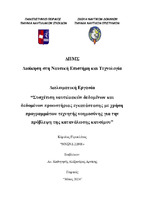
View/ Open
Keywords
Τεχνητή νοημοσύνη ; Μηχανική μάθηση ; Πρόβλεψη ; Κατανάλωση ; Πλοίο ; Artificial intelligence ; Machine learning ; Prediction ; Consumption ; ShipAbstract
The modern global shipping environment faces many challenges, most notably ongoing climate change and current geopolitical instability in regions surrounding major shipping routes. These situations are destabilizing factors for the global economy, causing significant disruptions in the global supply chain.
In addition to the economic impact of these situations on national economies, they also have a significant impact on the proper functioning of shipping companies, with the economic consequences often being extremely adverse.
Today’s shipping companies have to comply with increasingly stringent regulations imposed by the IMO, including the Safety of Life at Sea (SOLAS) and environmental targets to reduce the total amount of pollutants emitted by the shipping industry by 2050.
The development of modern technological applications and the rapid evolution of artificial intelligence are being integrated into the operations of shipping companies to make them sustainable and capable of achieving the IMO's goal.
The integration of artificial intelligence techniques allows shipping companies to analyze data from the fleets of ships they manage and draw reliable conclusions, that lead to optimal decisions. These decisions lead shipping companies to achieve their goals and economic growth, making the shipping industry a pioneer and leader in the global economy and in the fight against climate change.
This paper highlights the challenges that shipping companies face. The marine environment and its impact on ship operations are analyzed. Subsequently, artificial intelligence methods are extensively referenced and their application areas are analyzed, such as voyage planning, fuel consumption and failure prediction in the context of a ship.
Finally, the results of the development of four machine learning models for predicting the fuel consumption of a warship are analyzed, evaluated and compared. The aim of this study is not to select the best model for predicting fuel consumption, but to gain further knowledge that will help future research.