Η κανονική περίοδος και τα πλέι-οφς του NBA : σύγκριση και προβλέψεις με στατιστικές μεθόδους
Regular season and playoffs at the NBA : comparison and predictions using statistical methods
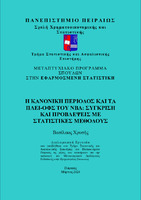
View/ Open
Keywords
NBA basketball ; Μπάσκετ ; Λογιστική παλινδρόμηση ; Μηχανική μάθηση ; Χρονοσειρές ; Στατιστική ανάλυσηAbstract
In modern times, data is considered an integral part of society, reflecting the digital world as well as our interactions within it. Data acquisition and, subsequently, its analysis leads to extraction of important information, shedding light to many “gray” aspects. From the pharmaceutical industry to sports, technology has contributed to the acquisition of a vast pool of data. More specifically in sports, particularly in basketball events, data analysis has fundamentally altered how teams and their coaches plan the games as well as how they behave on the field. With the continuous flow of statistical data for both players and teams, coaches get to have better insights and, consequently, improve the “Achilles Heel” of their team's and redefine their strategies. Thus, data analysis seems to have unlocked a whole new level of advanced preparation and competitiveness in championships.
This thesis focuses on sports and utilizes a combination of the performance indicators collected in National Basketball Association (NBA) games (e.g points per game and assists) and novel, recently created statistical variables, such as possessions and the DRt index. Initially, a descriptive analysis of all variables for the data collected from the last fifteen (15) seasons of the tournament is presented through tables and appropriate graphs. Then, certain tests of normality and correlation are conducted before applying suitable generalized linear models to find the most significant variables affecting championship conquest and a team's advancement (or lack thereof) to the Playoffs stage. Additionally, a time series data analysis is performed to provide forecasts for the next two (2) seasons. Subsequently, appropriate machine learning techniques are used to create classification models and explore if there are classes with common characteristics among the data (clustering) to examine team advancement to the Playoffs stage. The final conclusions of the study are presented along with comparisons to previous analyses conducted.