Εφαρμογές μηχανικής μάθησης στην νόσο του Alzheimer
Application of machine learning in Alzheimer disease
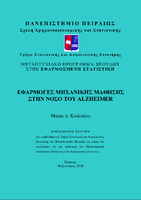
View/ Open
Keywords
Machine learning ; Alzheimer diseaseAbstract
Alzheimer's Disease (AD) is a type of dementia with particularly complex pathology and symptoms, and it is one of the most common causes of death in the elderly population. The identification of the disease in its early stages, before serious symptoms’ manifestation, is very important. The reason is that an appropriate intervention, as soon as possible, could slow down the progression of the disease. Mild Cognitive Impairment (MCI) can be an early stage because a significant proportion of individuals with MCI develop AD. This means that the diagnosis of this disorder against AD presents a great deal of interest. Nevertheless, the lack of a complete understanding of the mechanisms that cause and govern AD makes its diagnosis challenging, especially in the initial stages. In addition, common features with other types of dementia, cognitive disorders, or even other pathological conditions are obstacles to early detection. One way to overcome these challenges, as well as the subjectivity that characterizes a doctor's diagnosis, is to use machine learning models. This thesis focuses on the study and comparison of various machine learning techniques, aiming to identify AD versus MCI. The data includes demographic characteristics, scores on cognitive tests, and the volumes of 31 regions of interest in each hemisphere, extracted from MRI scans. The results of 10-fold cross validation show that the SVM classifier achieves the most accurate identification, compared to other classifiers. The model recorded an average accuracy rate of 92% and an average AUC of 94%. It is considered that the high performance is a result of the cognitive tests, since the diagnosis was based on MMSE, but it is enhanced by the MRI data.