Προβλέψεις αποδόσεων μετοχών του Ελληνικού Χρηματιστηρίου με τη χρήση μηχανικής μάθησης
Forecasting stock returns of the Greek Stock Exchange using machine learing techniques
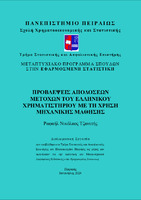
Προβολή/ Άνοιγμα
Λέξεις κλειδιά
Τεχνητή νοημοσύνη ; Θεωρία χρονοσειρών ; Αποδόσεις μετοχών ; Νευρωνικά δίκτυαΠερίληψη
Σε ένα κόσμο που κυριαρχεί η αβεβαιότητα και τα πάντα είναι ρευστά, η ανάγκη του
ανθρώπου για την δημιουργία ορθών προβλέψεων αποτελεί προτεραιότητα στην μείωση του
ρίσκου. Η δημιουργία νέων τεχνολογιών σε συνδυασμό με την αύξηση της υπολογιστικής
ισχύος, έδωσε νέα τροπή στην δημιουργία πιο εύστοχων προβλέψεων. Σε αυτή την
διπλωματική εργασία, θα παρουσιαστεί αρχικά το θεωρητικό υπόβαθρο γύρω από την ανάλυση
και την πρόβλεψη χρονοσειρών με κλασσικές μεθόδους. Πιο συγκεκριμένα, θα αναλυθούν τα
συνθετικά στοιχεία των χρονοσειρών, τα είδη των υποδειγμάτων που χρησιμοποιούνται,
διάφορα στατιστικά μέτρα, η ύπαρξη στασιμότητας, καθώς και διάφορες μέθοδοι πρόβλεψης
για κάθε τύπο προβλήματος. Στο δεύτερο κεφάλαιο, θα γίνει αναφορά στη μηχανική μάθηση,
στα είδη της, καθώς και στις διαφορές της σε σχέση με την βαθιά μάθηση. Παράλληλα, θα γίνει
αναφορά στον βιολογικό νευρώνα, θα παρουσιαστεί εκτεταμένα το θεωρητικό πλαίσιο και ο
τρόπος λειτουργίας τεσσάρων αρχιτεκτονικών τεχνητών νευρωνικών δικτύων και πιο
συγκεκριμένα, των LSTM, GRU, RNN και MLP. Επιπροσθέτως, θα παρουσιαστούν οι πιο
γνωστές συναρτήσεις βελτιστοποίησης, καθώς και συναρτήσεις απώλειας. Στο τρίτο κεφάλαιο,
θα δοθούν κάποια γενικά γνωρίσματα γύρω από τις αποδόσεις μετοχών, όπως επίσης και τρόποι
υπολογισμού τους. Στη συνέχεια, θα μελετηθούν οι λογαριθμικές αποδόσεις τριών εταιρειών
της Ελληνικής χρηματιστηριακής αγοράς και συγκεκριμένα του ομίλου της ΓΕΚ ΤΕΡΝΑ Α.Ε.,
της ΑΕΡΟΠΟΡΙΑΣ ΑΙΓΑΙΟΥ Α.Ε. και του ΟΠΑΠ Α.Ε., με την χρήση τεσσάρων
αρχιτεκτονικών νευρωνικών δικτύων και συγκεκριμένα των LSTM, GRU, RNN και MLP,
καθώς και έξι διαφορετικών αλγορίθμων βελτιστοποίησης. Ακολούθως, θα συγκριθούν βάσει
των πληροφοριακών κριτηρίων σφάλματος, όπου θα βρεθούν τα πιο αποδοτικά μοντέλα, ανά
δίκτυο. Κλείνοντας, θα διεξαχθεί η μετατροπή των προβλεπόμενων λογαριθμικών αποδόσεων
σε προβλεπόμενες τιμές κλεισίματος, τα αποτελέσματα των οποίων, θα απεικονισθούν σε
διαγράμματα.