Ανάλυση CT-Scans με χρήση συνελικτικών νευρωνικών δικτύων
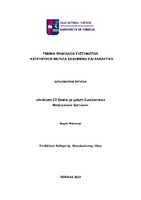
View/ Open
Keywords
Deep learning ; Image classification ; Συνελικτικά νευρωνικά δίκτυα ; 3D CNNs ; COVID-19 ; PyTorch ; TorchIOAbstract
In the period following the emergence and continuous spread of the COVID-19 disease, numerous cases and many deaths have been reported worldwide, each with varying degrees of impact in different countries. As a result of this situation, numerous PCR tests and medical image acquisitions (including CXRs and CT-Scans) are performed daily. Remarkably, healthcare systems globally are overwhelmed, leading to the infection of healthcare workers, even with the implementation of necessary safety measures. It would be particularly valuable to diagnose COVID-19 more quickly and accurately through CT-Scans, as it has been observed that the PCR diagnostic method lacks high sensitivity for mild COVID-19 infections.
Given that the interpretation of CT-Scans by specialized radiologists requires a significant amount of time, the development of an automated artificial intelligence system that operates more rapidly and with high accuracy would be extremely beneficial. The outcome of this development would be to alleviate the workload of specialists. Consequently, this would relieve the burden on both specialists and healthcare facilities and enable better control of COVID-19 cases. As a result, improved organization can be achieved, particularly in critical cases requiring immediate intensive care unit admission, as well as in mild cases suitable for home recovery.
This thesis statement presents and analyzes various types of Deep Learning algorithms, using axial tomography (CT-Scans), and demonstrates significant results with high accuracy. The current study focuses on the analysis of axial tomography images to categorize cases into COVID-19 patients and non-patients, thereby creating an automated system that could lead to the decongestion of healthcare systems and more efficient case management. In this work, different 3D Convolutional Neural Networks (CNNs) were constructed and appropriately trained following suitable image preprocessing techniques. Valuable results, conclusions, as well as graphical representations and images that aid in understanding the problem and comparing the results are presented.