Εφαρμογές της κατανομής Pareto σε δεδομένα αναλογισμού
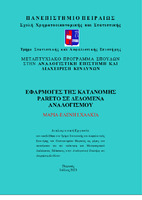
Προβολή/ Άνοιγμα
Λέξεις κλειδιά
Κατανομή ParetoΠερίληψη
Η παρούσα διπλωματική εργασία επικεντρώνεται στην κατανομή Pareto, τις μεθόδους εκτίμησης
των παραμέτρων της, καθώς και στην αξιολόγηση των εκτιμητών αυτών. Ο κύριος σκοπός της
εργασίας είναι η ανάλυση και η εξέταση της κατανομής Pareto με βάση πραγματικά δεδομένα,
καθώς και η προσαρμογή της κατανομής σε αυτά τα δεδομένα.
Αρχικά, γίνεται μια εισαγωγή στην κατανομή και η σημασία της σε διάφορους τομείς.
Εξετάζεται το θεωρητικό υπόβαθρο της κατανομής, συμπεριλαμβανομένου του ιστορικού, της
μαθηματικής μορφής, των μέτρων θέσης, διασποράς και ροπής, καθώς και της λοξότητας και
κύρτωσης. Επίσης, αναλύονται οι βασικές ιδιότητες της κατανομής Pareto και παρουσιάζονται οι
συναρτήσεις επιβίωσης και μέσης υπολειπόμενης ζωής.
Στο τρίτο κεφάλαιο, αναλύονται μια σειρά μεθόδων εκτίμησης των παραμέτρων της
κατανομής Pareto. Αρχικά, παρουσιάζεται η παραμετροποίηση της κατανομής, που περιλαμβάνει
τη μορφή της κατανομής και τις παραμέτρους της. Με τη χρήση αυτών των μεθόδων εκτίμησης,
είναι δυνατόν να εκτιμηθούν οι παράμετροι και να προσαρμοστεί η κατανομή στα παρατηρούμενα
δεδομένα.
Στο τέταρτο κεφάλαιο περιγράφονται λεπτομερώς τα μέτρα καλής προσαρμογής που
χρησιμοποιούνται για την αξιολόγηση των εκτιμητών και παρουσιάζονται οι στατιστικές
συναρτήσεις που χρησιμοποιούνται για τον υπολογισμό τους. Αυτά τα μέτρα περιλαμβάνουν τον
δείκτη Kolmogorov-Smirnov (KS), τον δείκτη Cramér-von Mises (CvM) και τον δείκτη
Anderson-Darling (AD). Κάθε μέτρο έχει τη δική του στατιστική συνάρτηση που χρησιμοποιείται
για τον υπολογισμό του και αποτελεί μια ποσοτική μέτρηση της προσαρμογής των εκτιμητών στα
δεδομένα Pareto. Η παρουσίαση αυτών των στατιστικών συναρτήσεων επιτρέπει την ακριβή και
αντικειμενική αξιολόγηση της απόδοσης των εκτιμητών.
Στο πέμπτο κεφάλαιο αναλύεται η δυσκολία των κατανομών να περιγράψουν πλήρως ένα
σύνολο δεδομένων σε όλο το εύρος τους. Υποστηρίζεται ότι οι κατανομές μπορούν να
περιγράψουν ικανοποιητικά τις μικρές ή μεσαίες τιμές, αλλά όχι τις μεγάλες. Παρουσιάζονται οι
γενικές μορφές των σύνθετων (composite) συναρτήσεων, ειδικότερα της εκθετικής και της Pareto,
καθώς και η σχετική μορφή της hazard.
Το έκτο κεφάλαιο επικεντρώνεται στους ευνοϊκούς εκτιμητές (favorable estimators) της
κατανομής Pareto. Αρχικά, γίνεται μια εισαγωγή στο θέμα και αναλύονται τα μέτρα καλής
προσαρμογής, τα οποία χρησιμοποιούνται για να αξιολογηθεί η προσαρμογή του μοντέλου στα
δεδομένα. Ένα σημαντικό μέτρο καλής προσαρμογής είναι το σημείο απώλειας, το οποίο
υπολογίζει το ποσοστό των παρατηρήσεων που πέφτουν έξω από την προσαρμοσμένη κατανομή.
Στη συνέχεια, εξετάζεται το κριτήριο ευρωστίας, το οποίο χρησιμοποιεί τη διακύμανση των
εκτιμητών ως ένα μέτρο της αστάθειας της εκτίμησης και παρουσιάζονται διάφοροι ευνοϊκοί
εκτιμητές της κατανομής. Αυτοί περιλαμβάνουν εκτιμητές ποσοστημορίων, εκτιμητές κομμένου
μέσου όρου και γενικευμένους εκτιμητές διαμέσων. Κάθε εκτιμητής έχει τις δικές του ιδιότητες
και περιορισμούς και χρησιμοποιείται ανάλογα με την εφαρμογή και τα δεδομένα που διαθέτουμε.
Στο έβδομο κεφάλαιο της διπλωματικής αναλύεται η εφαρμογή όλων των εκτιμητών σε
πραγματικά δεδομένα και παρουσιάζονται σημαντικά συμπεράσματα σχετικά με την απόδοση και
την ακρίβεια των εκτιμητών. Αρχικά, περιγράφεται η επιλογή του δείγματος και η μεθοδολογία
που ακολουθήθηκε για την αξιολόγηση των εκτιμητών. Εξηγείται ο τρόπος υπολογισμού των
εκτιμητών και παρουσιάζονται γραφήματα που απεικονίζουν την αρχική εκτίμηση προσαρμογής
των δεδομένων. Στη συνέχεια, παρουσιάζονται οι στατιστικοί έλεγχοι που πραγματοποιήθηκαν
και τα αποτελέσματα τους. Τέλος, γίνεται η κατάταξη των εκτιμητών βάσει της ανθεκτικότητάς
τους και της απόδοσής τους, προκειμένου να προκύψουν συγκεκριμένες συστάσεις για την
επιλογή του βέλτιστου εκτιμητή. Οι αναλύσεις και τα αποτελέσματα αυτά συνοψίζουν την
αξιοπιστία και την απόδοση των εκτιμητών και αποτελούν σημαντική συνεισφορά στην
κατανόηση του μοντέλου. Τα σχήματα, ο προσδιορισμός των εκτιμητών και οι αντίστοιχοι έλεγχοι
πραγματοποιήθηκαν με χρήση της Mathematica.