Δημιουργία συνθετικών νανοεπιφανειών με μεθόδους μηχανικής και βαθειάς μάθησης
Generation of synthetic nano-material surfaces through machine learning / deep learning methods
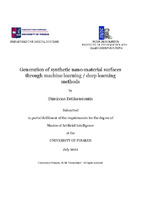
Master Thesis
Author
Δεληκωνσταντής, Δημήτριος
Delikonstantis, Dimitrios
Date
2022-07View/ Open
Keywords
Νανοεπιφάνειες ; Τραχύτητα ; Deep Convolutional Generative Adversarial Network ; N-Gram GraphsAbstract
Nano-technology is a branch of science that engages with engineering and technology performed on a nano-scale level. Nano-material rough surfaces are characterized by structural diversity and complexity. Nano-materials exhibit unique properties, such as friction, contact deformation and wettability, which is correlated to their surface roughness. Investigating the relationship between surface roughness and properties can lead to better nano-material designs and even to the discovery of new nano-materials with unique properties. In this research, we study the domain field of nano-roughness and nano-material surfaces as well as machine learning methods, with a focus on deep learning, in order to generate realistic synthetic nano-surface images, given specific surface roughness parameters. To this end we examine how prior domain knowledge can empower a model to provide realistic, synthetic surface images. We build upon previous work which was implemented by Vasileios Sioros in his research ”Generating Realistic Nanorough Surfaces Using an N-Gram-Graph Augmented Deep Convolutional Generative Adversarial Network”[1]. We contribute to the aforementioned work by introducing a new loss component to the network, reflecting the heights and frequency spectrum of nano-surface images. Furthermore, we propose a novel network architecture in an effort to reduce the checkerboard artifact observed in generated nano-surface images. Additionally, we optimize multi-component losses so that they equally contribute to the network’s learning process. We evaluate generated nano-surface images with quantitative measures. Also, a qualitative evaluation is carried out by a domain expert. In conclusion, our results are substantially improved compared to previous work’s results. The source code is available at https://github.com/ddelikonstantis/RoughML.