Improving human-robot collaborative reinforcement learning through probabilistic policy reuse
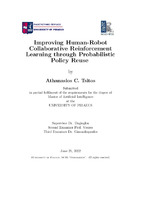
Master Thesis
Συγγραφέας
Τσίτος, Αθανάσιος Χριστόφορος
Tsitos, Athanasios C.
Ημερομηνία
2022-06-21Επιβλέπων
Δαγιόγλου, ΜαρίαDagioglou, Maria
Προβολή/ Άνοιγμα
Λέξεις κλειδιά
Socially aware robots ; Human-robot co-learning ; Deep reinforcement learning ; Soft actor-critic ; Transfer learning ; Probabilistic policy reuseΠερίληψη
Socially aware robots should be able, among others, to support fluent human-robot
collaboration (HRC) in tasks that require interdependent actions in order to be solved.
Similar to human-human collaboration, during HRC the actions of each agent affect
the actions of its partner. Towards enhancing mutual performance, collaborative robots
(cobots) should be equipped with adaptation and learning capabilities. Overall, mutual
learning can be a time consuming procedure that depends on the computational complexity of the task, the motor and cognitive load demanded, as well as the skills of the
human partner. Nevertheless, cobots should be able to integrate in their actions the
capabilities of their human partner and adapt to their strengths and weaknesses. In the
current thesis, we focused on HRC settings where a human and a Deep Reinforcement
Learning (DRL) agent need to learn in real-time how to solve a shared task through
efficient collaboration. In such scenarios, the performance of the team depends on one
hand on the ability of the DRL agent to learn how to solve the task while adapting to
its human partner and on the other hand on the ability of the human to understand
the strengths and weaknesses of the agent and adapt accordingly. The goal of the thesis
was to observe how the mutual performance could be improved when the agent needs to
collaborate with different humans. The method used was a transfer learning technique
called Probabilistic Policy Reuse, which allows DRL agents to take actions based on
other pre-trained policies. In order to access this method, we developed a human-agent
game where the human and a DRL agent controlled by the Soft Actor-Critic algorithm
needed to jointly control the motion of the end-effector of a robotic manipulator and
bring it to a goal position. For the experiments, we asked 16 different people to participate. Half of them played the game with a naive agent, meaning that the agent started
to play without having any knowledge about the game, while the other half played the
game with an agent, which had access to the actions of an expert agent that was trained
beforehand by the author. In the second group, the agent took actions based on his
current policy with a probability ψ and actions based on the expert policy with a probability 1ψ. The performance of the teams was evaluated through the travelled distance
of the end-effector and the results showed that there was a significant difference between
the performance of the teams which played without transfer learning and the teams
that played with. This result indicates that applying transfer learning in HRC scenarios
where the agent needs to collaborate with different humans might improve the mutual
performance of the team.