Μελέτη ακραίων παρατηρήσεων σε χρηματοοικονομικές χρονοσειρές με στοχαστική μεταβλητότητα
Extremes in financial time series with stochastic volatility
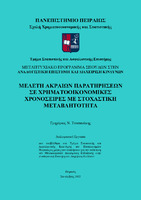
View/ Open
Keywords
Arch models ; Garch models ; Extreme value theory ; POT method ; Block Maxima method ; Peak Over Threshold method ; Financial Time Series with Stochastic Volatility ; Time Series ; VaR ; TVaR ; Αυτοπαλίνδρομα Μοντέλα Δεσμευμένης Ετεροσκεδαστικότητας ; Γενικευμένα Αυτοπαλίνδρομα Μοντέλα Δεσμευμένης Ετεροσκεδαστικότητας ; Θεωρία ακραίων τιμών ; Στάθμη απόδοσης ; Αξία σε κίνδυνοAbstract
The main aim of this Master Thesis is to present and numerically evaluate an improved methodology for the estimation of the Value at Risk (VaR and TVaR) of a financial asset (e.g. stock, stock market index, cryptocurrency, etc.) using an alternative model compared to the simpler market one. According to the simpler model logarithmic returns are assumed to be normally distributed with constant volatility. However, this assumption is violated most of the time, as suggested by the data obtained from the market. The alternative approach presented in this thesis, combines Generalized Autoregressive Conditional Heteroskedasticity models (GARCH) with Extreme Value Theory (EVT) to obtain better VaR estimates assuming volatility clustering and heavy tails for the distribution of the log-returns.
In the first chapter, the main characteristics of time series are presented and the ARCH and GARCH models are introduced along with the appropriate methods required for their exposition.
In the second chapter, Extreme Value Theory is introduced along with its fundamental probabilistic results. Also, the main two statistical methods, Block Maxima and POT are presented for the statistical analysis of extreme value data and the parameters estimation of GEV and GPD distributions respectively.
In the third chapter, GARCH models are combined with the Extreme Value Theory, in order to obtain improved estimates for VaR and TVaR.
In the fourth chapter, the aforementioned methodology is illustrated by applying the VaR and TVaR estimation GARCH-EVT methods to cryptocurrency data (Bitcoin). Furthermore, we back-test the proposed model using stock-market and cryptocurrency data (Amazon,Tesla,Bitcoin,Dow Jones Index,FTSE Index,Nasdaq Index,) and numerically assess its effectiveness against simpler models.
The practical application of the 4th Chapter was carried out using the R package, both for the statistical processing and the presentation of the results.