Τεχνικές εξόρυξης γνώσης στον τραπεζικό τομέα. Μελέτη περίπτωσης: πρόβλεψη πιθανότητας αθέτησης στις πιστωτικές κάρτες
Data mining techniques in the banking sector. Case study: predicting credit card default probability
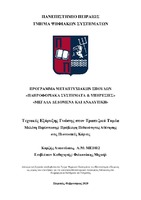
Προβολή/ Άνοιγμα
Λέξεις κλειδιά
Εξόρυξη δεδομένων ; Τραπεζικός τομέας ; Πιστωτικές κάρτες ; Πιθανότητα αθέτησης πιστούχουΠερίληψη
Στη σημερινή εποχή η τεχνολογία έχει αναπτυχθεί περισσότερο από κάθε άλλη επιστήμη. Σε αντίθεση με παλαιότερα, τις τελευταίες δεκαετίες, επικρατεί η τάση η έννοια τεχνολογία να αναφέρεται κυρίως στην τεχνολογία τον υπολογιστών. Στη σύγχρονη πραγματικότητα, κυριότερες ανάγκες και προκλήσεις στον τομέα αυτό αποτελούν, τόσο η διαχείριση του τεράστιου όγκου δεδομένων, τα οποία αυξήθηκαν εκθετικά – και εξακολουθούν να αυξάνονται – τα τελευταία χρόνια, όσο και η αξιοποίηση των δεδομένων αυτών με τον βέλτιστο τρόπο ώστε να παραχθούν πολύτιμες, αξιόπιστες και ενδεχομένως άγνωστες έως τώρα πληροφορίες. Τα πλεονεκτήματα από την επίτευξη των παραπάνω στόχων είναι ποικίλα και δύνανται να επηρεάσουν καταλυτικά πλήθος επιχειρηματικών κλάδων. Ο κλάδος των χρηματοοικονομικών είναι ένας από τους κυριότερους, και μάλιστα σε μια εποχή όπου η παγκόσμια και ευρωπαϊκή οικονομία βρίσκονται σε φάση αξιοσημείωτης επιβράδυνσης, προσπαθώντας παράλληλα να ανακάμψουν από την παγκόσμια οικονομική ύφεση του 2008 (μια από τις μεγαλύτερες στην ιστορία). Γίνεται εύκολα επομένως κατανοητό ότι ιδιαίτερα για τον τραπεζικό κλάδο, που αποτελεί την καρδιά της εκάστοτε οικονομίας, η χρήση εξελιγμένων μορφών τεχνολογίας μπορεί να βοηθήσει ουσιαστικά στην αυτοματοποίηση και βελτιστοποίηση των διαδικασιών με αποτέλεσμα αφενός τον περιορισμό των κινδύνων και αφετέρου την κερδοφορία.
Οι προαναφερθείσες έννοιες και οι μεταξύ τους σχέσεις παρουσιάζονται και αναλύονται στην παρούσα διπλωματική εργασία, η οποία στοχεύει στο να αποτυπώσει βασικές θεωρητικές γνώσεις επί των νέων τεχνολογιών και καινοτομιών και να τις συνδυάσει με τη σημασία τους για τον τραπεζικό κλάδο. Πιο συγκεκριμένα, η εργασία αρχικά (1ο και 2ο Κεφάλαια) πραγματεύεται τις έννοιες των Μεγάλων Δεδομένων, της Εξόρυξης Δεδομένων, της Μηχανικής Μάθησης και της χρήσης λύσεων Τεχνητής Νοημοσύνης στον επιχειρηματικό κόσμο. Έπειτα (3ο Κεφάλαιο) ο συντάκτης εστιάζει στη συσχέτιση των ανωτέρω με τον χρηματοπιστωτικό τομέα και ιδιαίτερα στη σχέση τους με την εκτίμηση και τον περιορισμό ενός από τους σημαντικότερους κινδύνους που αντιμετωπίζουν οι τράπεζες, τον πιστωτικό κίνδυνο. Η ανάλυση μάλιστα κατευθύνεται σταδιακά σε μια πιο εξειδικευμένη περιοχή, αυτή της εκτίμησης της πιθανότητας αθετήσεως πιστούχου για το χαρτοφυλάκιο των πιστωτικών καρτών, μιας κατηγορίας δανείων με ιδιαίτερα χαρακτηριστικά. Στα επόμενα δυο κεφάλαια που έπονται, πραγματοποιείται η πρακτική εφαρμογή αλγορίθμων μηχανικής μάθησης επί συνόλου δεδομένων τραπέζης εξωτερικού για την πρόβλεψη της πιθανότητας αθετήσεως πιστούχου και η αξιολόγηση των αποτελεσμάτων.
Η διαδρομή που καλείται ο αναγνώστης να ακολουθήσει στα πλαίσια της συγκεκριμένης εργασίας, περνώντας από το «γενικό» στο «ειδικό» και συσχετίζοντας διαφορετικές έννοιες - αλληλένδετες ωστόσο – είναι απόρροια τόσο του ενδιαφέροντος του συντάκτη επί των σχετικών αντικειμένων όσο και του ακαδημαϊκού και επαγγελματικού υπόβαθρού του.