A comparative evaluation of machine learning algorithms: binary classification on medical data
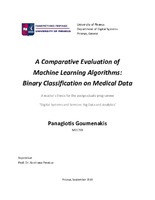
Master Thesis
Συγγραφέας
Γουμενάκης, Παναγιώτης
Goumenakis, Panagiotis
Ημερομηνία
2019-09Επιβλέπων
Πρέντζα, ΑνδριάναΠροβολή/ Άνοιγμα
Λέξεις κλειδιά
Machine learning algorithms ; Binary classification ; SVM ; Naïve Bayes ; Decision trees ; Logistic regression ; ANN ; Mesothelioma dataset ; UCIΠερίληψη
Στις μέρες μας ο τομέας της μηχανικής μάθησης έχει εφαρμοστεί και αναγνωριστεί ως ένα αποτελεσματικό εργαλείο που μπορεί να διαχειριστεί ένα ευρύ φάσμα πραγματικών καταστάσεων συμπεριλαμβανομένων και αυτών των ιατρικών εφαρμογών. Καθώς ο όγκος των δεδομένων στον τομέα της υγείας αυξάνεται χρόνο με το χρόνο, η εξέλιξη της πρόγνωσης μιας νόσου με τη χρήση εφαρμογών της μηχανικής μάθησης είναι αξιοσημείωτη. Οι εφαρμογές ακόμα της μηχανικής μάθησης στον τομέα της υγείας παρουσιάζουν ακριβή αποτελέσματα τόσο στην πρόβλεψη μίας επιδημίας ή διαφόρων ασθενειών όσο και στη συνεισφορά της βελτίωσης των τρόπων με τους οποίους σημειώνονται και αποθηκεύονται τα ιατρικά δεδομένα.
Αυτή η διπλωματική εργασία δίνει έμφαση αρχικά στην ανάλυση ιατρικών δεδομένων παρουσιάζοντας οπτικοποιήσεις αλλά και μετρικές σχετικά με τις πληροφορίες που παρουσιάζουν τα δεδομένα. Έπειτα, επικεντρώνεται στην υλοποίηση των κατάλληλων αλγορίθμων ικανών να ταξινομήσουν τα δεδομένα με σκοπό να καθορίσουν εάν ένας άνθρωπος έχει προσβληθεί από τη νόσο ή όχι. Η επιλογή της καταλληλότερης μεθόδου κρίνεται ως καθοριστικής σημασίας καθώς η εφαρμογή της σε πραγματικές καταστάσεις θα μπορούσε ενδεχομένως να βελτιώσει τόσο τις κλινικές αποφάσεις όσο και το προσδόκιμο ζωής του ασθενή.
Η συγκεκριμένη έρευνα βασίζεται στο σύνολο δεδομένων “Νόσος Μεσοθηλίωμα” που βρίσκεται στην αποθήκη συνόλων δεδομένων UCI και περιέχει 324 παρατηρήσεις με 35 χαρακτηριστικά. Σχετικά με τον τομέα της ανάλυσης που ασχολείται με τη μη επιβλεπόμενη μάθηση χρησιμοποιούνται αλγόριθμοι μηχανικής μάθησης για κατηγοριοποίηση όπως Δέντρα Απόφασης (Decision Trees), Μηχανές Διανυσμάτων Στήριξης (SVM), Λογιστική Παλινδρόμηση (Logistic Regression), k Πλησιέστεροι Γείτονες (kNN) και Νευρωνικά Δίκτυα (ΑΝΝ) με σκοπό να ολοκληρωθεί η ανάλυση και να οδηγηθεί κανείς σε αποτελέσματα και συμπεράσματα.
Όσον αφορά στις τεχνικές αξιολόγησης ο αναγνώστης μπορεί να περιμένει μεθόδους όπως για παράδειγμα τις στατιστικές μετρικές ακρίβεια (accuracy), ευαισθησία (sensitivity) και προσδιοριστικότητα (specificity), f1-score, την μήτρα σύγχυσης (confusion matrix) και τη χαρακτηριστική καμπύλη λειτουργίας (AUC/ROC).