Πειραματική μελέτη, αξιολόγηση και σύγκριση μεθόδων πρόβλεψης της οριακής τιμής συστήματος της ηλεκτρικής ενέργειας στην ελληνική αγορά ενέργειας
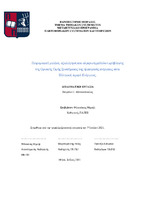
View/ Open
Keywords
Οριακή τιμή συστήματος ; Ελληνική αγορά ηλεκτρικής ενέργειας ; Μέθοδοι προβλέψεων ; Κυλιόμενη πρόβλεψηAbstract
The purpose of this Diploma Thesis is the implementation and evaluation of various models
for forecasting the System Marginal Price of electricity in the Greek Energy Market. Firstly,
the physical interpretation of the Energy System Marginal Price and its effect as an
indicator on the modern Energy market is presented. Then, an attempt is made to record the
various methods of forecasting the OTS and their separation into causal models and time
series models. In the next stage, a theoretical presentation of the metric errors was made,
which will then be calculated for each model in order to indicate the appropriate model for
the prediction in future data.
Having covered the theoretical background of this project, it is time to deal with the
practical part. The analysis was carried out with data from the RAE archive, to be more
specific with hourly OTS data from 01/01/2018 until 01/11/2020. After applying
appropriate transformations, these data were loaded into corresponding data structures and
examined as a time series model in terms of stationarity, seasonality and missing values
which have a theoretical explanation. Another attempt was made, this time, to estimate
multiple seasonalities of the time series object through the Fast Fourier Transform and these
seasonalities were used below. A number of methods were used and it was deemed
necessary to separate the forecasting process into long-term and short-term, since the
experimental conditions, data nature and especially data volume result to different handling
on a case-by-case basis. Regarding short-term prediction TBATS library was tested, ARMA
models from different libraries, ARIMA model superposition with Fourier components and
the FBProphet library. All the above models were trained in first 2 years data and tested in
the third year, using the last 2 methods in long-term forecast under different physical
conditions.
Finally, the corresponding metrics for each model were presented and based on them the
appropriate forecasting model was selected. As a result, in the last chapter the best model
trained with all existing data and future forecasting of OTS was attempted both in short and
long term, trying to extract knowledge and business value.