Ημιπαραμετρική συμπερασματολογία για μοντέλα επιταχυνόμενων χρόνων ζωής
Semiparametric inference for accelerated life test models
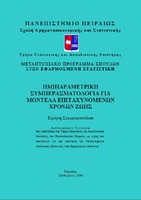
View/ Open
Keywords
Accelerated life test models ; Density ratio models ; Distribution (Probability theory) ; Statistical analysis ; Bootstrap (Statistics)Abstract
Accelerated life time models are used in order to faster estimate life distributions of
systems without waiting to observe their lifetimes under normal operating conditions. These
models appeared many decades ago and are applied mainly in the areas of biostatistics and
mechanical engineering. The purpose of this dissertation is to introduce the reader to this kind
of models and to apply a semiparametric method of estimating the life time distribution.
In the first chapter we start with a description of accelerated life test models by
commenting on their usefulness and we continue with basic definitions of notions which are
used in the dissertation.
In the second chapter we present the density ratio model introduced by Fokianos et al.
(2001) based on ideas of Qin and Lawless (1994) and we explain in detail the estimation
process of its parameters via profiling the (nonparametric) likelihood. Based on the estimates
of the parameters we estimate the unknown distribution function. The method is applied to a
real data example.
In the third chapter we discuss the density ratio model by further modeling its
parameters. The simpler model is compared to the basic one asymptotically and using the
bootstrap method.
In the fourth chapter we consider the density ratio model in the case where the data are
subject to (possible) random censoring. In this case our estimation procedures are based on a
particular EM algorithm recently proposed by Wei and Zhou (2016).
For the estimation of the models and all other calculations we used R and more
specifically the function optim. The procedures which return the loglikelihood and its
derivatives are presented in the fourth chapter.
We conclude by discussing some questions and thoughts as well some ideas for future
work.