Πρόβλεψη χρηματιστηριακών δεικτών με τεχνικές εξόρυξης δεδομένων σε υβριδικές πηγές δεδομένων
Prediction of stock market indices with data mining techniques in hybrid data sources
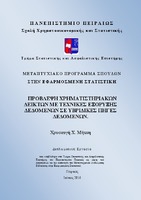
View/Open
Keywords
Χρονοσειρές ; Εξόρυξη δεδομένων ; Χρηματιστήριο Αξιών Αθηνών ; Προβλέψεις ; Χρηματιστηριακοί δείκτεςAbstract
Nowadays, time series prediction, especially in the case of financial time series, is undoubtedly a matter of widespread research activity. Indeed, in finance, time series analysis is applied widely not only for the purposes of predicting prices of international and national markets but also it is used for the prediction of financial crises or / and investment strategies.
It is also a fact that modern decision support systems are based on information extracted from structured data. So, by neglecting the unstructured data may provoke the loss of significant information. This, in combination with the rapid development of technology has led to the need for new dynamic tools that will help transform unstructured data into structured information, which when combined with information extracted from structured data will help investments to make the best decision. A new field of science, called data mining, is going to supply that need. It is about a combination of different sciences such us statistics, machine learning, information theory and computational procedures.
In this thesis we introduce an innovative data processing system in order to predict the trend of banking shares of Athens Stock Exchange for the year 2014. Therefore, are examined three applications of the system in different data sets (arithmetic, text and hybrid) and then are evaluated so as to identify the more effective one. In this system are applied classification and clustering techniques in categorical data while presented the most basic concepts and methods used by classification, clustering and prediction techniques in data mining.
The aim of this thesis is by explaining data mining methods, to demonstrate not only its usefulness in finance but also its significance for drawing meaningful conclusions from unstructured and difficult in use data.